Kindly fill up the following to try out our sandbox experience. We will get back to you at the earliest.
AI Data Governance: Mastering Data Lineage & Management
Discover how AI-driven data governance enhances your data management with robust data lineage, ensuring data quality and integrity in your enterprise.
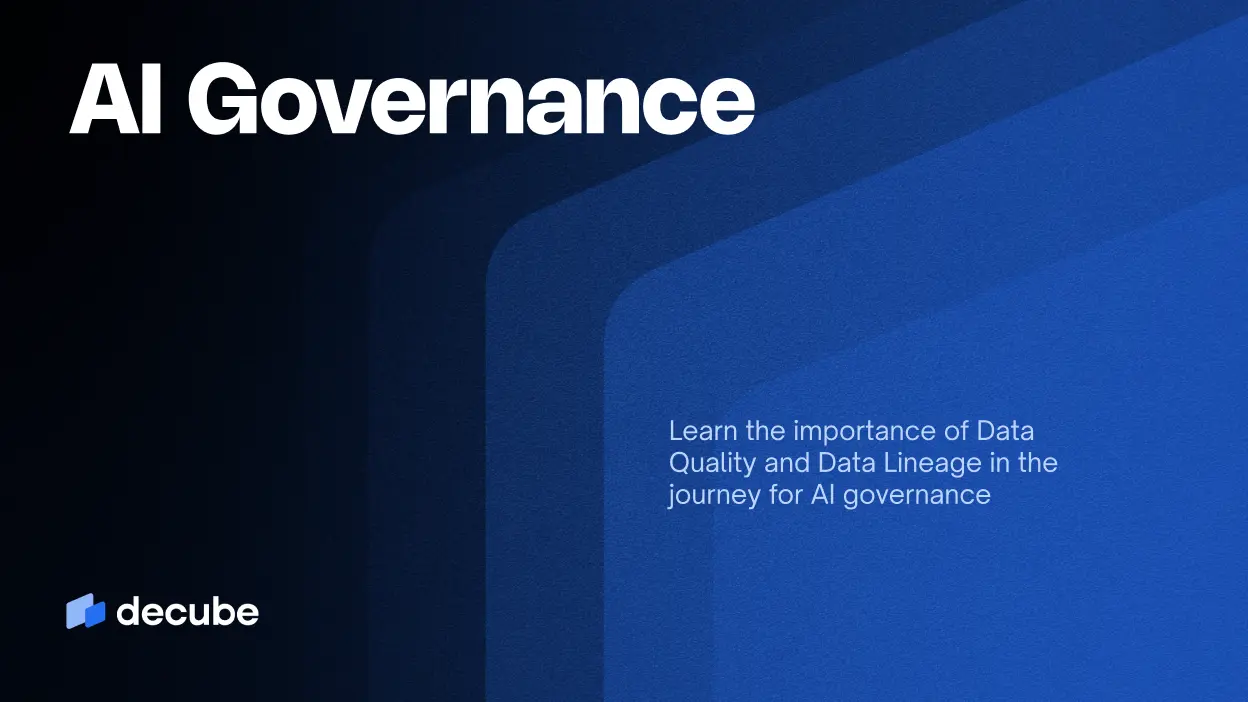
Did you know that over 80% of organizations struggle with data governance due to the complexities of managing data in the AI era? This fact highlights the need for strong AI data governance frameworks. These frameworks improve data quality and make sure it follows the law. By using advanced data lineage, companies can track where their data comes from, how it changes, and where it ends up. This builds trust in data and helps make better decisions at all levels.
The world of AI data governance is changing fast. Big names like Gartner, McKinsey & Company, and Forrester Research talk about how important metadata management is. Knowing about these areas is key for companies wanting to excel in data governance in today's digital world.
Key Takeaways
- AI data governance is key for keeping data safe and following the law.
- More than 80% of companies have trouble with data governance.
- Data lineage tracks the data's path from start to end.
- Metadata management is crucial for good data governance.
- Strong frameworks can make data better overall.
Understanding Data Lineage
Data lineage is key in data governance. It tracks data from its start to where it ends in an organization. This helps keep data quality high and follow laws. Knowing about data lineage is vital for finding and combining data from different places.
Managing metadata well is important too. With metadata, companies can see how data moves through their systems. This helps them handle data questions and problems better. Tools for tracking data lineage make it easier to see how data changes and moves, which helps with combining data.
Data lineage does more than just track data. It gives companies the insights they need for better data management. This leads to better decisions and more efficient operations.
Key Components of Data Lineage in AI Governance
Data lineage is key in managing data with AI. It ensures data quality and accuracy, which are crucial for good decision-making.
Key components of data lineage include:
- Data Provenance: This tracks where data comes from. It helps check data quality and reliability.
- Transformation Processes: These show how data changes over its lifecycle. Knowing these changes is important for keeping data quality high.
- End-User Access: Watching who can see the data keeps it secure and in line with governance rules.
Data lineage tools are essential for managing metadata well. They help visualize data flows, making it easier to follow governance rules. These tools also help keep an eye on data quality, fixing issues early.
By using strong metadata management, organizations can boost their data lineage strategies. This makes things more transparent and helps stakeholders make informed choices with reliable data.
Implementing Column Level Data Lineage
Column level data lineage is key to better data management in companies. It shows how data changes over time in detail. This helps organizations understand how data is used, leading to better analysis and decisions.
Using column level data lineage also improves data catalogs. It makes finding data easier and keeps data management strong. This way, companies can keep a tight grip on their data.
Tools like Decube make it easier to use column level data lineage. They help with data governance by keeping track of all changes. Companies that use this method get ahead and make their data management smoother. It builds trust and openness around their data.
Managing Data with AI
AI is key in helping organizations manage their data better. It makes data governance stronger. With AI tools, companies can automate tasks like data management. This ensures data quality stays consistent.
AI helps with integrating data from different sources. This is a big help for companies with lots of data. It makes reporting and analytics easier.
AI is great at finding problems early in the data process. This means better data quality. It helps make data processing more efficient.
AI uses predictive analytics and machine learning to help make better decisions. This makes companies work smarter. AI also builds trust and transparency in how data is managed.
Best Practices for AI Data Governance
Effective AI data governance needs to follow best practices that match the company's goals. It's key to have clear data lineage processes. This makes data handling transparent and traceable. It builds trust among stakeholders and helps make consistent decisions.
Good data management means using data quality metrics to keep getting better. By checking these metrics often, companies can see how well their data work is doing. This helps improve data quality and spot areas to get better at.
It's vital to build a culture that values data stewardship and accountability in AI data governance. When every employee knows their part in keeping data quality high, data use becomes more responsible. Training programs that teach about data lineage and quality are key. They keep everyone updated on the latest best practices and standards.
- Align governance strategies with business objectives
- Implement data lineage processes for transparency
- Utilize data quality metrics for improvement
- Foster a culture of data stewardship
- Provide ongoing training and awareness programs
By following these best practices, companies can get better at AI data governance. This supports strong data management.
Wrap up
Understanding data lineage is key in AI data governance. It helps improve data quality and manage it better. This article showed how important strong data lineage frameworks are.
Decube offers solutions for taking data governance further. Booking a demo or consultation shows how their tools can improve data quality and meet AI needs. By focusing on data governance, companies can make big strides in their operations and goals.
FAQ
What is AI data governance and why is it important?
AI data governance is about making sure data is good quality and follows rules. It uses AI to help with this. It's key for making smart choices and following laws.
How does data lineage contribute to data management?
Data lineage shows where data comes from and where it goes. This keeps data reliable and in line with rules. It helps find and combine data better, making data management better.
What are data lineage tools and why are they necessary?
Data lineage tools show how data moves and changes in systems. They are key for keeping track of data details, making data better, and following data rules.
What are the key components of data lineage in AI governance?
Important parts include knowing where data comes from, how it changes, and who can see it. These help keep data reliable and support in managing data details, which is key for data rules.
How can column-level data lineage improve data management practices?
Tracking data changes at a detailed level with column-level lineage gives deeper insights. This makes data catalogs more accurate and helps manage data details better.
What role does AI play in managing data?
AI does routine tasks, makes data better, and helps combine data smoothly across different systems. This boosts how things work and helps with data rules.
What are the best practices for establishing AI data governance?
Good practices include matching governance with business goals, using strong data lineage, promoting data care, and keeping up with training in data governance.
How can organizations ensure data quality and integrity?
Companies can keep data good by using strong data governance with a focus on lineage, setting quality goals, and good metadata management.
What is the relationship between data discovery and metadata management?
Data discovery needs good metadata management for context and structure. This makes it easier for companies to find and use important data.