Kindly fill up the following to try out our sandbox experience. We will get back to you at the earliest.
Data Governance : What is it? Concepts, Benefits and Comprehensive Guide
Unlock the power of data governance: Learn fundamentals and strategies for becoming truly data-driven.
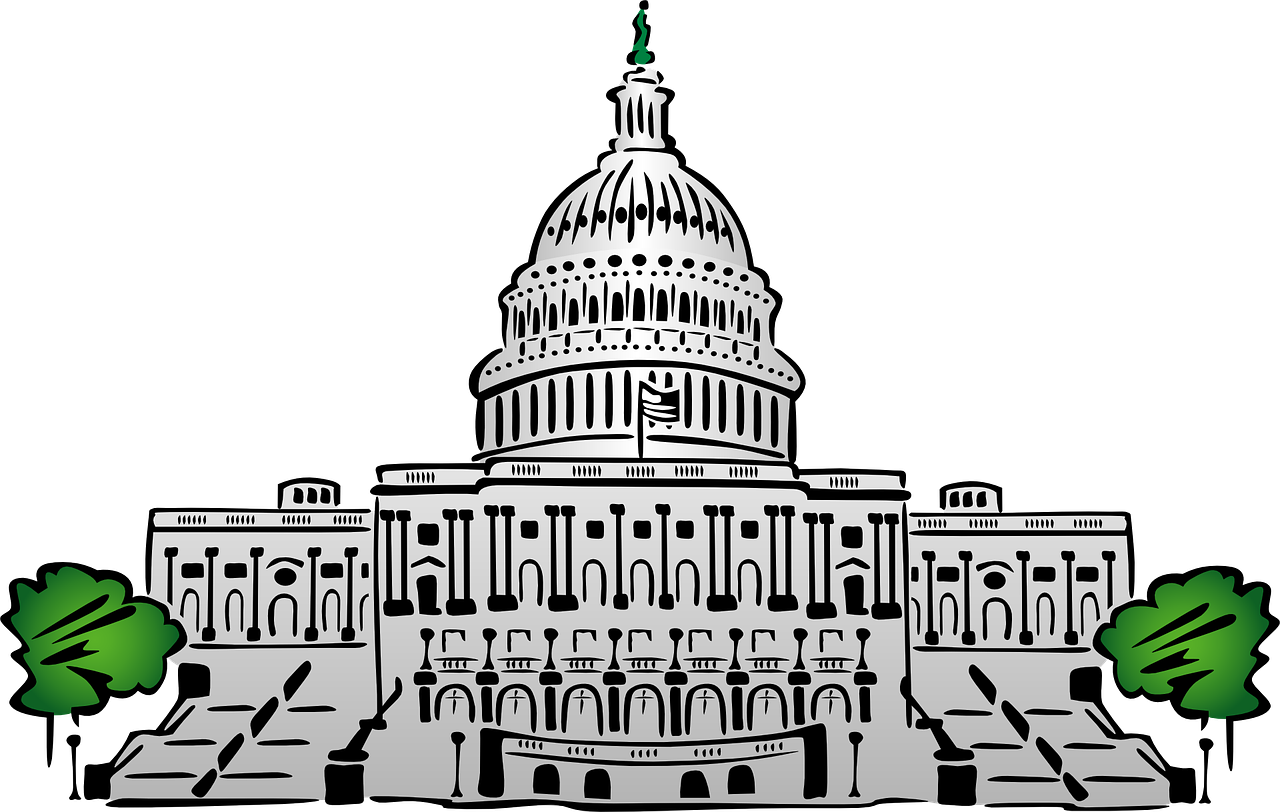
What is Data Governance?
Making your company truly data-driven
Disclaimer: This article is not written using Chatgpt :)
I never imagined that my journey as a data engineer would eventually lead me to start a data observability company. But as I look back on my experiences, I see how gaining exposure to data has been a constant thread throughout my career, guiding me to where I am today. Serendipity is not just a fancy word but also a satisfying feeling!
As a data guy, at the beginning of my career, I focused solely on building systems to collect and process data. However, handling sensitive information was always tricky — a fact every person working in data can empathize with. I realized that simply having the technology to collect data, build ETL pipelines, and then use data for modeling & prediction was of no use when data governance principles were not enforced within an organization.
My team and I would spend long exhausting hours troubleshooting issues where the culprit would mostly be “bad data”.
Key Takeaways
- Data governance is crucial for organizations to make informed decisions, improve cost controls, enhance regulatory compliance, and earn trust from stakeholders.
- It involves setting internal standards and complying with external regulations to ensure data accuracy, quality, privacy, and compliance.
- Data governance enables organizations to manage risk, provide more access to data, and make data-driven decisions.
- Implementing data governance in the cloud requires addressing data security, regulatory compliance, and visibility and control.
- Key components of data governance include data stewardship, data quality management, data discoverability, and metadata management.
“Data is unreliable, data is missing, data is inaccurate’ was a common dialogue for us.”
Key Features of Data Governance:
Data Life Cycle: Data governance encompasses the entire life cycle of data, including its creation, storage, usage, and disposal.
Data Policies: Internal standards in the form of data policies and guidelines that govern data access, usage, and management within an organization.
Internal Standards: Establishing frameworks and processes to ensure consistency in data management practices across the organization.
External Standards: Compliance with industry best practices and legal requirements set by industry associations and government agencies.
Ensuring that data is ethically and securely in accordance with industry regulations was and is of importance in every organization. Effective data governance ensures that data is consistent and trustworthy and doesn’t get misused. And being a dreamer, I always dreamt of a super simple platform that helps data engineers solve data quality issues at a lightning speed.
This is where my interest in data governance or observability grew multifold. I learned that data governance was more than just data security — it was about ensuring that data was used in a responsible and controlled manner throughout its lifecycle. In fact, I have seen the consequences of poor data governance, such as data breaches leading to business loss.
The overall concept of data governance may sound very basic but it’s often overlooked especially when companies are just starting out. Apart from failing to build a data infrastructure, they often overlook the data security aspect of their business as well.
The importance of data governance has only increased with the rise of new technologies. As a strong advocate for healthy data governance practices, I envision a future where data is used responsibly and ethically, to the benefit of both businesses and their customers.
Why you need Data Governance?
If we go by the book’s meaning of it, data governance is a set of processes and policies that govern the collection, storage, use, and dissemination of an organization’s data.
Let’s go one by one on what data governance means:
- Data Quality Management: Data needs to be accurate, consistent, and free of errors. For example, an organization may implement data quality checks to validate that customer addresses are correctly formatted before they are stored in the company’s database.
- Data Security and Privacy: Implement measures to protect data from unauthorized access and breaches. Plus, ensure you’re complying with privacy regulations such as the General Data Protection Regulation (GDPR).
- Data Retention and Disposal: Establish policies for how long data should be kept and when it should be deleted or disposed of, in accordance with legal requirements and best practices. In short, it’s all about adding rules to the stored data.
- Data Access and Usage: Define who has access to data and how it can be used, in line with the organization’s data governance policies. For example, a company may restrict access to sensitive customer data to only those employees who have a valid business reason for using it.
The implementation of effective data governance policies and procedures helps organizations to avoid the costly consequences of poor data management, such as data breaches and loss of customer trust. It can also help to ensure regulatory compliance and promote the responsible and ethical use of data.
But why do we need data governance?
Companies have faced massive fines and damage to their reputation due to data breaches and the misuse of customer information.
Here are a few occurrences:
In 2018, a marketing and data aggregation company based in Florida called Exactis made a major mistake that had far-reaching consequences. They left a database with personal information on nearly 340 million people on a server that anyone could access. This information included people’s phone numbers, addresses, email addresses, interests, and details about their families, including the number, age, and gender of their children.
Thankfully, a security expert discovered the breach and brought it to the public’s attention. We are talking about millions of adults’ and businesses’ data being exposed. This example highlights the importance of proper data governance and the serious consequences that can occur when personal information is not properly secured.
By now you must have understood the importance of data governance. It’s undoubtedly relevant to all companies, regardless of size or industry. Every company collects and uses data in some way, and without proper governance, this data is at risk of being misused, lost, or stolen. In addition, new regulations like the General Data Protection Regulation (GDPR) and the California Consumer Privacy Act (CCPA) require companies to be more transparent about how they use personal data. So if you are in a company or have a company, there’s no escape from enforcing good data practices. Either the hackers or the Government will be knocking on your door when it gets too late. And I personally wouldn’t wish that on anyone.
Use Cases of Data Governance
Data governance plays a crucial role in various aspects of data management, ensuring accountability, data quality, and discoverability. Let's explore the key use cases where data governance provides significant value:
Data Stewardship
Data governance empowers organizations to assign accountability and responsibility for data to data stewards. Data stewards act as custodians, overseeing the proper handling, usage, and governance of data. By establishing data stewardship roles and responsibilities, organizations can ensure data is managed effectively throughout its lifecycle.
Data Quality
Data governance is instrumental in maintaining data quality standards. By implementing data governance practices, organizations can establish standardized data quality rules, perform consistent data profiling and cleansing activities, and enforce data validation and verification processes. This promotes the integrity and reliability of data used for decision-making and operational processes.
Data Management
Data governance plays a significant role in managing data as a valuable enterprise asset. It ensures that data is managed consistently and effectively across the organization, aligning with data management best practices. Through data governance, organizations establish data policies, procedures, and frameworks that facilitate efficient data integration, storage, and retrieval, promoting data efficiency and accuracy.
Data Discoverability
Effective data governance enables organizations to enhance data discoverability, making it easier to find and access relevant data assets. By establishing metadata management practices, organizations can create comprehensive data catalogs that provide detailed information about data assets, facilitating efficient search and discovery. Improved data discoverability enables teams to make informed decisions, leverage data insights, and enhance collaboration.
Metadata Management
Data governance promotes effective metadata management, ensuring the consistent and accurate documentation of metadata across the organization. Metadata, such as data definitions, data lineage, and data classifications, helps users understand the context, quality, and usage of data assets. By implementing metadata management strategies, organizations can enhance data governance practices and enable more efficient data stewardship and data management processes.
By leveraging data stewardship, ensuring data quality, managing data effectively, enabling data discoverability, and implementing proper metadata management, organizations can harness the full potential of data governance and achieve data-driven success.
Data Governance and Data Management
Data governance and data management are two interconnected concepts that play crucial roles in managing and leveraging an organization's data assets. While data governance focuses on establishing policies and standards for data use, data management encompasses the broader scope of managing data throughout its lifecycle, ensuring its quality, availability, and usability.
Data governance is the framework that defines how data is controlled, protected, and consumed within an organization. It involves establishing data governance councils or committees, defining data governance principles, and implementing processes to ensure adherence to data policies and standards. The goal of data governance is to enable effective decision-making, mitigate risks, and ensure data compliance with regulatory requirements.
Data management, on the other hand, encompasses the operational aspects of handling data. It involves activities such as data capture, storage, integration, analysis, and archival. Data management focuses on implementing the necessary technologies, processes, and practices to ensure data quality, accuracy, and reliability. It also includes data stewardship, which assigns accountability and responsibility for data to specific individuals or teams.
Effective data governance relies on robust data management practices to ensure the integrity and usability of data. Here are some key areas where data governance and data management intersect:
- Data Quality: Both data governance and data management initiatives prioritize data quality to ensure that data is accurate, consistent, and reliable. Data governance establishes quality standards and processes, while data management puts those standards into action through data profiling, cleansing, and validation techniques.
- Metadata Management: Metadata, which provides context and meaning to data, is a critical component of both data governance and data management. Data governance defines and manages metadata standards, while data management ensures the appropriate collection, storage, and usage of metadata throughout the data lifecycle.
- Data Ingestion: Data governance and data management collaborate to establish robust data ingestion processes. Data governance ensures that data being ingested aligns with defined policies and standards, while data management handles the technical aspects of data ingestion, including data integration, transformation, and loading.
To better understand the relationship between data governance and data management, refer to the table below:
Challenges of Data Governance
Data governance encounters various challenges due to the complex nature of data management and evolving data landscapes. Several factors impede the effectiveness of data governance initiatives, requiring organizations to address these obstacles strategically.
Data Silos and Integration
Data silos pose a significant challenge to data governance efforts. In many organizations, data is scattered across different systems, applications, and departments, resulting in fragmented data landscapes. These silos hinder data integration and the ability to gain a holistic view of the organization's data assets. Data integration becomes essential to unify diverse data sources and ensure accurate and comprehensive data analysis.
Data Engineering Problems
Data governance faces unique data engineering problems, such as data quality issues, data transformation complexities, and data lineage tracking. Data engineers play a crucial role in implementing data governance practices and overcoming these challenges. They must ensure the availability, accessibility, and reliability of data through efficient data engineering processes.
Evolving Data Use Cases
As the use of data continues to evolve, organizations face the challenge of adapting their data governance frameworks to address emerging data use cases. New business requirements, technological advancements, and changing regulations introduce unprecedented data management scenarios that demand agile and adaptable governance strategies. Organizations must continuously evaluate and update their data governance practices to accommodate these evolving use cases.
In order to overcome these challenges, organizations must establish standardized tool usage to streamline data governance processes. Additionally, acknowledging the human side of data governance is crucial. This involves fostering a culture of data stewardship, where individuals take ownership and are accountable for data governance. Finally, implementing best practicesrecommended by industry experts can help organizations optimize their data governance strategies and achieve success.
Addressing these challenges enables organizations to establish efficient and effective data governance practices, ensuring data integrity, compliance, and maximizing the value of their data assets.
Best Practices for Data Governance
Implementing effective data governance requires following best practices that enhance the overall management and control of data. These practices include:
- Leveraging Existing Metadata: Utilize metadata sharing to maximize the value of existing data and streamline data governance processes.
- Starting Small and Focused: Begin by implementing data governance initiatives on a smaller scale, focusing on specific areas or departments. This approach allows for easier management and adoption, ensuring a smoother transition towards comprehensive data governance.
- Change Management Techniques: Implement Change Management techniques to facilitate smooth adoption and acceptance of data governance practices within the organization. This includes effective communication, stakeholder involvement, and training programs.
- Implementation Steps: Define clear goals and objectives for the data governance program and derive a roadmap for its implementation. This ensures a systematic and organized approach, minimizing disruptions and maximizing the program's success.
- Data Governance Framework: Develop a structured data governance framework that brings together people, technology, and processes. A robust framework provides a clear structure for data governance activities, establishes accountability, and ensures consistent compliance with data governance principles and policies.
Implementing these best practices will enable organizations to establish a strong foundation for data governance, enhance data management practices, and achieve data-driven decision-making. By leveraging existing metadata, starting small, using change management techniques, following implementation steps, and creating a data governance framework, organizations can ensure the success and effectiveness of their data governance programs.
Components of a Data Governance Framework
A data governance framework consists of various components that collectively form the foundation of a successful governance program. These components encompass policies, rules, processes, organizational structures, and technologies aimed at ensuring effective data management and control.
The key components of a data governance framework are:
- Policies: Governing policies establish the guidelines and principles for data governance within an organization. These policies address data handling, privacy, security, and compliance to ensure consistency and standardization.
- Rules: Data governance rules are specific guidelines that are followed to enforce data policies. These rules define the procedures and processes for data management, data access, data quality, and data lifecycle management.
- Processes: Data governance processes encompass the activities and workflows involved in managing data throughout its lifecycle. These processes include data classification, data stewardship, data integration, data security, and data validation.
- Organizational Structures: The organizational structure of a data governance framework defines roles, responsibilities, and reporting lines within an organization. This structure ensures clear accountability and governance oversight, with designated data governance teams, data stewards, and data owners.
- Technologies: Employing suitable technologies is essential for effective data governance. These technologies include data governance platforms, metadata management tools, data cataloging tools, and data quality management solutions. These technologies automate governance processes, enable collaboration, and provide visibility into data assets.
- Mission Statement: A clear mission statement establishes the purpose and objectives of data governance. It outlines the organization's commitment to data integrity, compliance, and effective data management practices.
- Data Catalogs: Data catalogs provide a centralized repository of data assets, allowing users to discover, understand, and access relevant data. These catalogs document data lineage, data definitions, data usage policies, and data access permissions, facilitating the organization's overall data governance efforts.
Implementing a data governance framework with well-defined policies, rules, processes, organizational structures, technologies, and a mission statement creates a solid foundation for effective data governance practices. By leveraging the right components, organizations can ensure data consistency, integrity, security, and compliance throughout their data lifecycle.
Rounding Up
Effective data governance goes beyond data observability and cataloging tools. It also encompasses master data management, data asset ownership, and decision-making processes. Master data management ensures that the organization's core data, such as customer information or product data, is accurate, consistent, and reliable across systems. Data asset ownership involves assigning responsibility for data quality and ensuring that data is treated as a valuable asset. Additionally, data governance should include processes for making data-driven decisions, ensuring that stakeholders have access to reliable and trustworthy data to inform their choices. By incorporating these elements into your data governance strategy, you can ensure the integrity, reliability, and value of your data assets.
In the era of data-driven decision-making, effective data governance is crucial to ensure data accuracy, security, and ethical use. By implementing policies and procedures, organizations can protect against data breaches and maintain customer trust while complying with regulations like the General Data Protection Regulation (GDPR) and the California Consumer Privacy Act (CCPA) [1][2]. In countries like Australia, the United States, and South Korea, data governance is essential for businesses to prevent costly consequences and protect sensitive information [3][4]. Data observability tools, such as Monte Carlo, Databand, and Acceldata, can help improve data management efficiency and reliability. Start thinking about data governance today to safeguard your company and customers. Decube is among the few platform to manage data observability, catalog and governance.
[1] https://gdpr.eu/[2] https://oag.ca.gov/privacy/ccpa[3] https://www.oaic.gov.au/privacy/australian-privacy-principles/[4] https://www.ftc.gov/tips-advice/business-center/privacy-and-security
FAQs:
General:
- What is Data Governance?
Data Governance is the set of policies, processes, and tools that ensure the availability, accuracy, security, and usability of an organization's data throughout its lifecycle, from creation to retirement. It's essentially managing your data like a valuable asset, making sure it's reliable and fit for purpose.
- Why is Data Governance important?
Data Governance delivers a wealth of benefits, including:
- Improved data quality: Consistent definitions, standards, and validation processes ensure your data is accurate and usable.
- Enhanced compliance: Data Governance helps you adhere to industry regulations and data privacy laws like GDPR and CCPA.
- Better decision-making: Trustworthy data leads to more informed and effective business decisions.
- Increased efficiency: Standardized data access and processes save time and resources.
- Reduced risk: Mitigates risks associated with data breaches, errors, and inconsistencies.
- Who is involved in Data Governance?
Data Governance is a collaborative effort involving various stakeholders:
- Data Owners: Business leaders responsible for defining data usage and ensuring compliance.
- Data Stewards: Subject matter experts who oversee specific data sets and implement governance practices.
- Data Users: Everyone who accesses and uses data within the organization.
- IT Department: Provides technical support and infrastructure for data management.
- What are the key components of a Data Governance program?
Effective Data Governance programs have these key components:
- Policies: Define data ownership, access, usage, security, and retention standards.
- Processes: Establish workflows for data creation, collection, storage, and access.
- Tools & Technologies: Software and platforms to automate tasks, manage metadata, and enforce policies.
- Roles & Responsibilities: Clear definition of roles and responsibilities for stakeholders.
- Metrics & Monitoring: Track progress, measure success, and identify areas for improvement.
- How does Data Governance differ from Data Management?
Data Management refers to the technical aspects of collecting, storing, and processing data. Data Governance focuses on the overall governance and control of that data, ensuring its quality, security, and compliance. Think of Data Management as the "how" and Data Governance as the "why" and "what" of data handling.
Implementation:
- How do I get started with Data Governance in my organization?
Start by:
- Assessing your current data landscape: Identify data sources, usage patterns, and potential issues.
- Defining your goals and objectives: What do you want to achieve with Data Governance?
- Developing a governance framework: Establish policies, processes, and roles.
- Building awareness and buy-in: Educate stakeholders about the importance of Data Governance.
- Starting small and scaling up: Implement Data Governance in phases, focusing on high-impact areas first.
- What are the common challenges faced in implementing Data Governance?
Common challenges include:
- Resistance to change: Users may be hesitant to adopt new processes.
- Lack of resources: Allocating budget, personnel, and time for Data Governance initiatives.
- Data silos: Breaking down departmental data barriers and establishing collaboration.
- Inconsistent data practices: Standardizing data definitions and formats across the organization.
- What tools and technologies can support Data Governance?
Many tools and technologies can aid Data Governance, including:
- Data Catalogs: Centralized repositories for metadata and data lineage information.
- Data Quality Tools: Identify and correct data errors and inconsistencies.
- Access Control Software: Manage user access to data based on permissions and roles.
- Data Encryption Tools: Secure sensitive data at rest and in transit.
- Workflow Automation Platforms: Automate data governance tasks and processes.
- How can I measure the success of my Data Governance program?
Track key metrics like:
- Data quality: Error rates, completeness, consistency.
- Compliance adherence: Meeting regulatory requirements.
- User adoption: Percentage of users following governance practices.
- Time to insights: Reduced time to extract valuable insights from data.
- Cost savings: Improved efficiency and reduced risks through data governance.
Advanced:
- How can Data Governance help me comply with data privacy regulations?
Data Governance plays a crucial role in data privacy compliance by:
- Identifying and classifying sensitive data: Knowing what data requires protection.
- Implementing access controls: Limiting access to authorized personnel only.
- Enforcing data retention policies: Retaining data for the required period and disposing of it securely.
- Enabling data subject rights management: Facilitating individual data access and deletion requests.