Kindly fill up the following to try out our sandbox experience. We will get back to you at the earliest.
Data Lineage Best Practices
Explore crucial data lineage best practices to boost data governance, ensure compliance, and uphold data quality across your organization's information ecosystem. Strengthen trust in your data with effective lineage strategies

Data Lineage Best Practices
"The goal is to turn data into information, and information into insight." - Carly Fiorina, former CEO of Hewlett-Packard
In the AI era, knowing where data comes from and where it goes is key. This is called data lineage. It's vital for good data management and governance. As data grows, following its path helps keep it accurate, follow rules, and make smart choices.
Data lineage shows how data moves, changes, and is used. It helps businesses improve their data handling, quality, and trust. Let's see why it's important for managing data well and how it adds value to businesses.
Key Takeaways
- Data lineage is crucial for effective data governance and management
- Implementing best practices enhances data quality and compliance
- Tracking data flow improves decision-making processes
- Data lineage builds trust in organizational data assets
- Understanding data journey is key to maintaining data integrity
Understanding Data Lineage Fundamentals and Its Strategic Importance
Data lineage is key to managing data today. It follows data from start to finish, through all changes and uses. This is vital for businesses to use their data wisely.
Defining Data Lineage in Modern Enterprise
In today's world, data lineage shows how data moves through systems. It reveals how data changes and who sees it. This is essential for keeping data quality high and making trustworthy business decisions.
Key Components of Data Lineage Architecture
A strong data lineage framework has several parts:
- Data sources and destinations
- Transformation rules and logic
- Data flow mapping
- Metadata management
Together, these parts give a clear view of data's journey and growth.
Business Value and ROI of Data Lineage Implementation
Data lineage offers more than just meeting rules. It boosts business value by:
- Improving data quality by tracking it better
- Making it quicker to see the effects of changes
- Less time lost on data problems
- Better decisions with reliable data
Companies that focus on data lineage see big gains in efficiency and data trustworthiness.
"Data lineage is not just about tracking data; it's about understanding the story our data tells and ensuring that story is accurate and valuable."
Essential Elements of a Data Lineage Framework
A strong data lineage framework is key to good data governance. It tracks data from start to finish, ensuring everything is clear and accountable. This is crucial for managing data well.
- Metadata management systems
- Data flow mapping tools
- Impact analysis techniques
- Automated lineage discovery
- Data quality monitoring
Metadata management is very important in a data lineage framework. It involves collecting, storing, and organizing data information. This helps teams understand data, its connections, and changes.
Data flow mapping is also crucial. It shows how data moves through systems. This helps spot problems and improve data flow.
Using these elements in a data lineage framework is a best practice. It gives organizations a solid base for data management. This helps them make smart choices, follow rules, and keep data quality high.
Data Lineage Best Practice: Implementation Guidelines
It's key to follow data lineage best practices for good data governance. Let's look at important steps to make it work in your company.
Establishing Data Lineage Standards
Make sure you have clear data lineage standards. This means setting rules for metadata, how to map data flows, and naming things. Also, create a template for recording data lineage info the same way everywhere.
Documentation Requirements and Protocols
Make detailed plans for documenting data lineage. List what you need to document, like where data comes from, how it's changed, and where it goes. Also, use version control to see changes over time.
Stakeholder Alignment and Communication
Get stakeholders involved early in setting up data lineage. Hold regular meetings to make sure everyone is on the same page. Also, have a plan to keep everyone updated on data lineage changes.
By sticking to these steps, companies can build strong data lineage practices. This improves data governance and makes managing data more efficient.
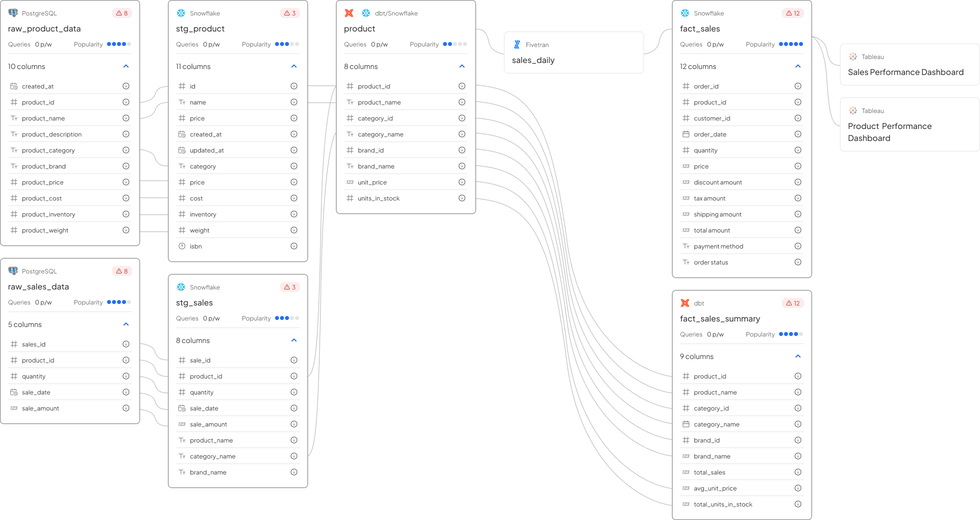
Integrating Data Lineage with Metadata Management
Data management is better when data lineage and metadata work together. This mix helps with data governance and shows how data moves in an organization.
Metadata Capture and Classification
It's key to capture and sort metadata to know where data comes from and how it changes. Data lineage tools help by automatically pulling out metadata. They look through databases, files, and apps to find important data details.
Building Metadata Repositories
A central metadata repository is vital for managing data lineage and metadata. It holds info on data sources, changes, and how it's used. With a detailed repository, companies can:
- Keep track of data changes
- Find data connections
- Follow data rules
- Boost data quality
Automated Metadata Discovery Solutions
Data lineage automation has changed how we find metadata. New tools use AI to study data flows and pull out metadata on their own. This cuts down on manual work and makes data lineage mapping more accurate.
By linking data lineage with strong metadata management, companies get a better view of their data world. This helps data experts make smart choices, keep data quality high, and follow rules.
Data Lineage Tools and Technology Selection
Choosing the right data lineage tools is key for good data management. These tools track data flow, ensuring it's accurate and follows rules. When picking data lineage visualization software, think about what your organization needs and what systems you already use.
- Automated data discovery
- Real-time lineage tracking
- Integration with existing systems
- Customizable visualization options
- Scalability for growing data volumes
Look at how well tools can handle your data, how easy they are to use, and if they meet legal needs. Many data lineage visualization platforms offer free trials. This lets you try them out before deciding to buy.
The best data lineage tool for your organization depends on your unique data landscape and goals. Take time to compare different options. Also, involve important people in the decision-making process.
Data Quality Control Through Lineage Tracking
Data lineage tracking is key to keeping data quality high. It helps us understand where data comes from. This way, we can manage and ensure data quality better.
Quality Metrics and Monitoring
Good data quality starts with clear metrics. These metrics track data's accuracy, completeness, and consistency. Data lineage lets us watch these metrics at every step.
This helps us catch quality problems early. It stops them from spreading through the system.
Impact Analysis and Change Management
Data lineage shows us how data is connected. This is vital for understanding changes' impacts. It helps us see how changes might affect data quality.
This knowledge helps us manage changes better. It lowers the risk of bad outcomes.
Data Quality Remediation Strategies
When quality issues pop up, data lineage is a big help. It shows us where and why problems happen. With this info, we can fix issues right at the source.
This not only solves current problems but also stops them from happening again. It leads to lasting data quality improvements.
Using data lineage in our data management is a smart move. It builds a strong system for keeping data quality high. This makes our data reliable and trustworthy, helping us make better decisions.
Automated Data Lineage Solutions and Benefits
Data lineage automation is changing how companies handle their data. These advanced tools make tracking data easier and help with better data management.
AI-Powered Lineage Discovery
AI tools for data lineage use machine learning to map data flows automatically. They scan big databases, find connections, and make detailed maps with little human help. This gives a clear view of how data moves in an organization.
Real-time Lineage Tracking Systems
Real-time data lineage tracking systems give instant insights into data flows. They watch data changes as they occur, helping spot problems fast. This is key for businesses that need accurate data quickly for making decisions.
Integration with Existing Data Infrastructure
Today's data lineage tools easily fit with current data systems. They work well with data warehouses, ETL tools, and analytics platforms. This gives a complete view of the data world.
Using these automated data lineage solutions helps companies manage their data better. It improves data quality and gives deeper insights into their data world. These tools do more than just make things more efficient. They help build a culture of trust and compliance with data.
Regulatory Compliance and Data Lineage Documentation
In today's world, keeping up with regulations is key for businesses. Data lineage documentation is vital for this. It tracks data from start to finish, showing a company's dedication to data quality and governance.
Good data lineage documentation helps companies in many ways:
- It proves data is accurate and reliable.
- It spots risks in how data is handled.
- It makes audits easier.
- It boosts data quality overall.
Laws like GDPR and CCPA demand detailed records of data use. Data lineage documentation makes it simple to follow these rules by showing how data moves through systems.
"Data lineage is the backbone of regulatory compliance in the digital age."
To stay compliant, companies should:
- Use strong data lineage tools.
- Keep documentation up to date.
- Train staff on data governance.
- Do regular audits of data processes.
By focusing on data lineage documentation, businesses can keep their data quality high. They can also handle the complex world of regulations with confidence.
Wrap Up
Data lineage best practices are key for good data governance and management. They help track data from start to finish. This ensures everything is clear and accountable in the data journey.
A strong data lineage framework is essential for businesses. It keeps data quality high, meets regulations, and aids in making smart decisions. It lets people see how data moves, spot problems, and fix them fast.
Using data lineage tools and automated solutions makes data management easier. It saves time and boosts data trustworthiness. This approach is efficient and reliable.
As data gets bigger and more complex, focusing on data lineage is more important. Companies that invest in solid data governance strategies will face data challenges better. They will achieve long-term success.
FAQ
What is data lineage and why is it important?
Data lineage tracks data from start to finish, showing all changes it goes through. It's key for managing data well, keeping it accurate, and following rules. It helps make better decisions by showing where data comes from and how it's used.
What are the key components of a data lineage framework?
A good data lineage framework has several parts. It includes managing metadata, mapping data flows, and analyzing impacts. It also has rules for capturing, classifying, and storing data. These parts give a full picture of data's journey in an organization.
How can organizations integrate data lineage with metadata management?
To link data lineage with metadata, use methods for capturing and classifying metadata. Build detailed metadata repositories and use tools for finding metadata automatically. This helps understand data better, making data management and quality control more effective.
What should be considered when selecting data lineage tools?
When picking data lineage tools, look at features like automation and visualization. Check if they work with your current systems and if they're easy to use. They should also handle complex data and support rules and regulations.
How does data lineage contribute to data quality control?
Data lineage helps track data, find errors, and improve quality. It lets you see how data changes and where problems might start. This way, you can keep data reliable and accurate.
What are the benefits of automated data lineage solutions?
Automated solutions make tracking data easier and faster. They reduce mistakes and give real-time views of data. AI can find complex data links, making lineage more accurate and complete.
How does data lineage documentation support regulatory compliance?
Documentation is key for following rules by showing data's journey. It proves data handling is done right, keeps data safe, and answers audits quickly. It's vital for meeting GDPR, CCPA, and other standards.
What are some best practices for implementing data lineage?
Start with clear rules and detailed records. Make sure everyone knows about it. Use automation and link it with data management. Keep it up to date and focus on quality and value.