Kindly fill up the following to try out our sandbox experience. We will get back to you at the earliest.
Data Mesh: A Paradigm Shift in Managing Data at Scale
Explore Data Mesh, its workings, benefits, and challenges in transforming data management at scale. Discover the future of data
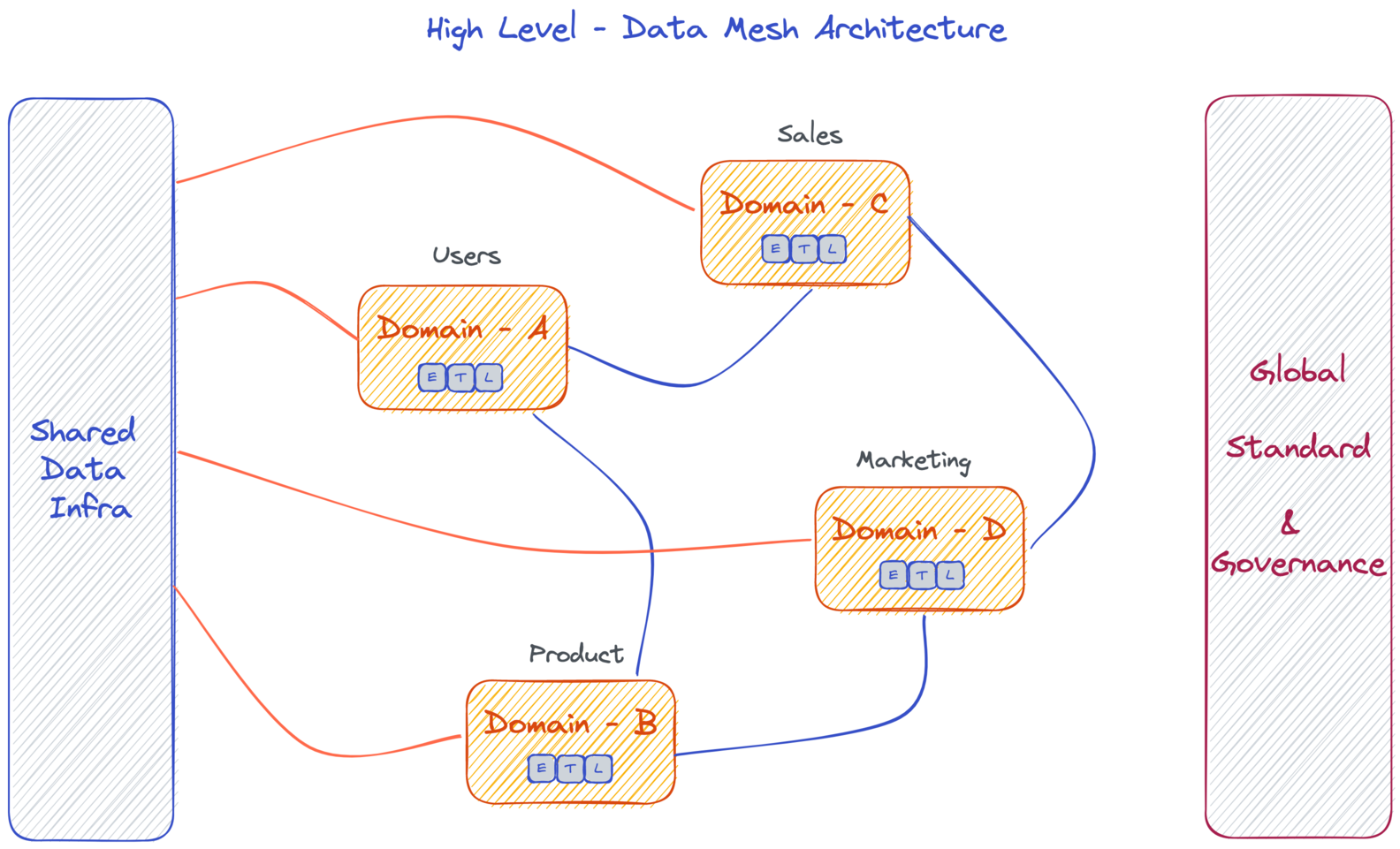
To provide a comprehensive understanding, we'll showcase real-world examples of organizations successfully leveraging Data Mesh to unlock their data's full potential. Stay tuned for an insightful journey into the world of Data Mesh!
What is Data Mesh?
Data Mesh is a new approach to data management that challenges the traditional centralized architecture. It promotes a decentralized and domain-oriented model, where each domain takes ownership of its own data products. This innovative technology enables organizations to scale their data infrastructure while fostering collaboration and empowering teams to make better use of their data resources. With Data Mesh, businesses can achieve more efficient and scalable solutions for managing their diverse datasets in the digital age.
The Traditional Approach to Data Management
Centralized data architecture, data silos, and lack of collaboration are inherent characteristics of the traditional approach to data management. In this model, all data flows through a central system controlled by the IT department. This leads to bottlenecks and delays in accessing and analyzing critical information. Additionally, different departments often maintain their own separate databases or "data silos," which hinder cross-functional collaboration and create redundant efforts. As a result, organizations heavily rely on their IT department for all data-related tasks, limiting agility and scalability within their technology ecosystem.
The traditional approach to data management is outdated, characterized by centralized control and data silos. However, the concept of data mesh offers a revolutionary alternative that decentralizes architecture and promotes collaboration across departments, leading to greater agility in managing enterprise-wide information assets.
In today's digital age where technology constantly evolves, this traditional approach is becoming increasingly outdated. The concept of data mesh offers a revolutionary alternative that decentralizes data architecture while promoting collaboration across departments through self-serve technologies and products. By adopting this new paradigm, organizations can break down silos, empower teams with autonomous decision-making capabilities over their respective domains of expertise without compromising overall governance requirements—leading to greater agility in managing enterprise-wide information assets effectively.
The Concept of Data Mesh
The concept of Data Mesh revolutionizes data management by adopting a decentralized approach, empowering domain-oriented teams to take ownership of their data. This shift in mindset treats data as a product, enabling better collaboration and innovation across the organization. With this architecture, technology meshes together to create autonomous data products that serve specific business needs.
Key points:
- Decentralized approach to data management
- Domain-oriented teams taking ownership of their data
- Treating data as a product mindset
How Does Data Mesh Work?
Domain-Oriented Decentralization: Data mesh is built on the principle of domain-oriented decentralization, where each domain team takes ownership of their own data products. This approach ensures that data is managed and governed by those who have the most context and expertise in a particular area.
Product-Oriented Self-Serve Platforms: In data mesh, self-serve platforms are created to enable domain teams to independently manage and serve their own data products. These platforms provide tools for collecting, processing, storing, and serving data in a way that is tailored to the specific needs of each domain.
Federated Computational Governance: Data mesh incorporates federated computational governance, which means that decision-making power is distributed across multiple teams rather than being centralized. This allows for more agility and adaptability in managing data as it evolves over time.
Data as a Product: With data mesh, organizations treat their internal datasets as products that can be consumed by different users or teams within the company. This shift in mindset encourages better documentation, versioning, discoverability, and accessibility of data throughout the organization.
Domain-Oriented Decentralization
Domain-Oriented Decentralization is a key principle of the Data Mesh approach, revolutionizing data management in the digital age. This approach focuses on empowering cross-functional domain teams to take ownership and accountability for their own data products. It also emphasizes decentralized data governance, allowing each domain team to manage and govern their data according to their specific needs.
- Cross-functional domain teams
- Autonomous ownership and accountability
- Decentralized data governance
Product-Oriented Self-Serve Platforms
Data product development platforms enable organizations to build and deploy data products at scale, empowering users with valuable insights. These platforms provide a self-serve environment equipped with intuitive tools and infrastructure that enable users to easily access, analyze, and visualize data. By adopting a product-oriented approach in data management, organizations can leverage the power of self-service platforms to drive innovation and make informed decisions based on real-time data analysis.
Federated Computational Governance
Distributed decision-making processes enable diverse stakeholders to actively participate in governing computational systems. By distributing decision-making power, organizations can tap into a wider range of expertise and perspectives, leading to more inclusive and effective governance. Collaborative governance models further enhance this by fostering collaboration between different entities, such as businesses, government agencies, and communities. These models promote shared responsibility and collective problem-solving for the development and regulation of computational systems. Additionally, embracing the 'code as law' principle ensures that algorithms are designed with legal compliance in mind, enhancing transparency and accountability within federated computational governance frameworks.
Data as a Product
Treating data as a valuable asset is essential in the digital age. By recognizing its potential for generating insights and driving business outcomes, organizations can unlock the true value of their data. Defining clear metrics for data product success allows companies to measure the impact of their data initiatives and make informed decisions based on quantifiable results. Incorporating feedback loops into the data product lifecycle ensures continuous improvement and adaptation, allowing organizations to evolve with changing market needs.
- Data as a valuable asset: Recognizing the significance of data in driving business outcomes.
- Clear metrics for success: Establishing measurable criteria to assess the effectiveness of data products.
- Feedback loops: Continuously refining and improving data products through iterative feedback mechanisms.
Benefits of Data Mesh
1. Unparalleled data quality and accessibility: By implementing a Data Mesh framework, organizations can ensure high-quality and easily accessible data. The decentralized approach enables each domain team to take ownership of their data, leading to improved data governance, accuracy, and reliability.
2. Accelerated speed and agility: With Data Mesh, companies experience increased speed in processing and analyzing large volumes of information. By distributing the responsibility for data products to individual teams, decision-making processes become more streamlined, enabling faster response times to market changes or emerging trends.
3. Fostered collaboration and autonomy: Embracing a Data Mesh architecture promotes collaboration between different domains within an organization. It encourages cross-functional teams to work together on specific areas of expertise while granting them the autonomy they need to innovate freely.
4. Seamless scalability and flexibility: One of the key benefits of adopting a Data Mesh model is its ability to scale effortlessly as businesses grow or evolve over time. The distributed nature allows for easy integration with new technologies or systems without disrupting existing workflows or compromising performance.
These advantages illustrate how implementing a Data Mesh strategy revolutionizes data management practices in today's digital age by improving data quality and accessibility while increasing speed, fostering collaboration among teams autonomously, all underpinned by scalable flexibility.
Improved Data Quality and Accessibility
Data governance and quality control are critical components of a data mesh framework. By implementing robust processes and systems, organizations can ensure that data is accurate, reliable, and consistent. This ensures improved data quality for decision-making purposes.
With easier data discovery and access, organizations can break down silos and enable seamless sharing of information across teams. This not only enhances collaboration but also accelerates insights generation by eliminating time-consuming manual searches.
Centralized metadata management allows for efficient tracking and organization of vital information about the available datasets. By maintaining a comprehensive repository, organizations can easily locate relevant datasets without wasting valuable time searching through multiple sources.
Increased Speed and Agility
Faster time to insights is essential in today's fast-paced digital landscape. With the implementation of a data mesh architecture, organizations can leverage real-time data processing capabilities to extract valuable insights instantaneously. This enables businesses to make informed decisions and stay ahead of the competition. Furthermore, the ability to quickly adapt to changing business needs is crucial for success in this dynamic environment. By embracing a data mesh approach, companies can easily scale their operations and adjust their strategies as required, ensuring agility and responsiveness in an ever-evolving market.
Enhanced Collaboration and Autonomy
Cross-functional teams are now empowered with data ownership, allowing them to take control of their own data assets and make informed decisions. This has resulted in increased collaboration between domain experts and engineers, fostering a more cohesive and efficient working environment. Furthermore, the reduced dependencies on centralized data teams have allowed for quicker decision-making processes and enhanced autonomy within each team's operations.
Scalability and Flexibility
Elastic scaling optimizes the allocation of infrastructure resources, allowing for seamless integration of new data sources and technologies. This modular architecture simplifies maintenance tasks, resulting in enhanced scalability and flexibility for efficient data management in the digital age.
Challenges of Implementing Data Mesh
1. Organizational Mindset Shift: Adopting a data mesh approach requires a fundamental change in the way organizations think about data management. Traditional centralized models are replaced with decentralized, self-serve teams, which can be met with resistance and reluctance to relinquish control.
2. Technical Complexity: Implementing a data mesh involves integrating multiple disparate systems and technologies, which can pose technical challenges. Ensuring seamless communication and coordination between these different components may require significant investments in infrastructure and expertise.
3. Data Governance and Security: With distributed ownership of data comes the challenge of maintaining consistent governance practices across various teams. Establishing clear guidelines for access control, privacy protection, compliance, and quality assurance becomes crucial to ensure trustworthiness and mitigate potential risks.
Organizational Mindset Shift
Decentralized ownership of data fosters agility and empowers teams to make data-driven decisions. Cross-functional collaboration and communication break down silos, enabling seamless knowledge sharing across departments. Shifting from a project-based to a product-based approach encourages continuous innovation and adaptability in the ever-evolving digital landscape. Embracing these organizational mindset shifts is crucial for successfully implementing a data mesh framework and revolutionizing data management in the modern era.
Technical Complexity
- Data infrastructure scalability: Building a scalable data infrastructure is crucial in the digital age, as organizations generate vast amounts of data. It requires careful planning and investment in robust systems that can handle increasing volumes of data without compromising performance.
- Data discovery and cataloging challenges: With diverse data sources and formats, it becomes challenging to discover relevant datasets and effectively catalog them for easy access. This complexity calls for advanced metadata management solutions to ensure efficient data discovery.
- Integration of diverse data sources: Integrating data from various sources with different structures, encoding schemes, or even languages poses significant technical complexities. Effective integration strategies need to be deployed to enable seamless flow of information across disparate systems.
Stay tuned for the next sub-heading where we delve into the critical aspect of Data Governance and Security in implementing a successful Data Mesh framework.
Data Governance and Security
Establishing clear data governance policies is crucial to effectively manage and protect data in the digital age. By defining rules and processes for data management, organizations can ensure consistency and accountability across all departments. This includes implementing measures to ensure data quality and accuracy, as well as facilitating seamless access to reliable information for decision-making purposes. Additionally, robust security measures must be put in place to safeguard sensitive data from unauthorized access or breaches.
- Establish clear guidelines for data management
- Ensure consistent quality of data across the organization
- Implement strong security measures for protecting sensitive information
Real-World Examples of Data Mesh
Netflix, one of the pioneers in implementing a data mesh approach, decentralized its data infrastructure by creating domain-oriented platform teams. This shift allowed individual teams to take ownership of their specific domains and responsibilities, resulting in increased autonomy and faster decision-making processes. By adopting this data mesh model, Netflix has been able to scale its operations while maintaining flexibility and agility.
LinkedIn also embraced the principles of a data mesh by reorganizing its data infrastructure into smaller autonomous units called “data product teams.” These cross-functional teams are responsible for end-to-end ownership of their respective products, including data pipelines and analytics services. This approach has empowered LinkedIn to deliver customized insights to its users more efficiently while promoting collaboration among different business units.
Uber's adoption of a data mesh framework revolutionized how it manages and utilizes large volumes of complex datasets. By transitioning from monolithic centralized systems to smaller decentralized ones known as "domain-oriented distributed architectures," Uber improved scalability and reduced bottlenecks in processing massive amounts of real-time streaming data. This transformation enabled Uber to provide more accurate ride estimations, optimize driver routes, enhance user experiences, and ultimately disrupt the transportation industry.
These real-world examples demonstrate how companies like Netflix, LinkedIn, and Uber have successfully implemented a data mesh strategy to overcome challenges associated with managing vast amounts of heterogeneous data sources. Through decentralization, domain ownership empowerment is achieved along with enhanced scalability capabilities for improved customer experiences within various industries
Netflix
Netflix has successfully implemented Data Mesh in its content recommendation system, revolutionizing the way personalized user experiences are enabled. By leveraging a decentralized approach to data infrastructure, Netflix has been able to scale their operations efficiently and effectively. With Data Mesh, they have unlocked new levels of accuracy and relevance in content recommendations, ensuring that users receive tailored suggestions based on their unique preferences and viewing habits.
Through the implementation of Data Mesh at Netflix, the traditional challenges associated with scaling data infrastructure have been overcome. This innovative approach allows for greater autonomy and ownership within cross-functional teams responsible for managing different aspects of the data ecosystem. As a result, Netflix is able to handle massive amounts of data seamlessly while maintaining high quality standards and minimizing bottlenecks. The adoption of Data Mesh sets a new standard for scalability in the digital age by distributing responsibility across teams and empowering them to make better decisions based on insights derived from their respective domains.
Improving data quality and accuracy is vital in today's digital landscape. With the implementation of Data Mesh, organizations can revolutionize their data management processes. By decentralizing data ownership and empowering teams with self-serve access to data, companies can ensure higher levels of accuracy and reliability in their analytics capabilities. The Data Mesh approach enhances collaboration among teams, leading to improved insights and decision-making based on reliable data sources.
Uber
Optimizing ride matching algorithms using a Data Mesh architecture has been instrumental in enhancing the efficiency and accuracy of Uber's services. By decentralizing data ownership and enabling real-time decision-making, Uber has created a dynamic ecosystem that ensures seamless integration of external partners' data. This implementation adheres to the principles of a Data Mesh, empowering Uber to deliver an unparalleled experience to its customers while maintaining robust data management practices.
Conclusion
Implementing a data mesh brings several benefits to organizations in the digital age. It enables scalability and agility, allowing teams to work independently and take ownership of their data domains. This decentralized approach promotes better collaboration and innovation while ensuring data quality and reliability.
However, adopting a data mesh approach also comes with its share of challenges and considerations. Organizations must carefully evaluate their existing infrastructure, processes, and culture to ensure a smooth transition. They need to invest in proper governance frameworks, establish clear communication channels, and provide adequate training for teams involved in managing the distributed architecture.
Overall, embracing a data mesh can revolutionize how organizations manage their data assets effectively. By empowering individual teams while maintaining centralized oversight, businesses can unlock new insights from their vast amounts of information while staying nimble in an ever-evolving digital landscape.