Kindly fill up the following to try out our sandbox experience. We will get back to you at the earliest.
Data Observability Use Case: Financial Industry
Explore how data observability enhances financial operations, ensuring regulatory compliance and risk management. Learn key use cases in Data Observability, Use Case in Financial Industry.
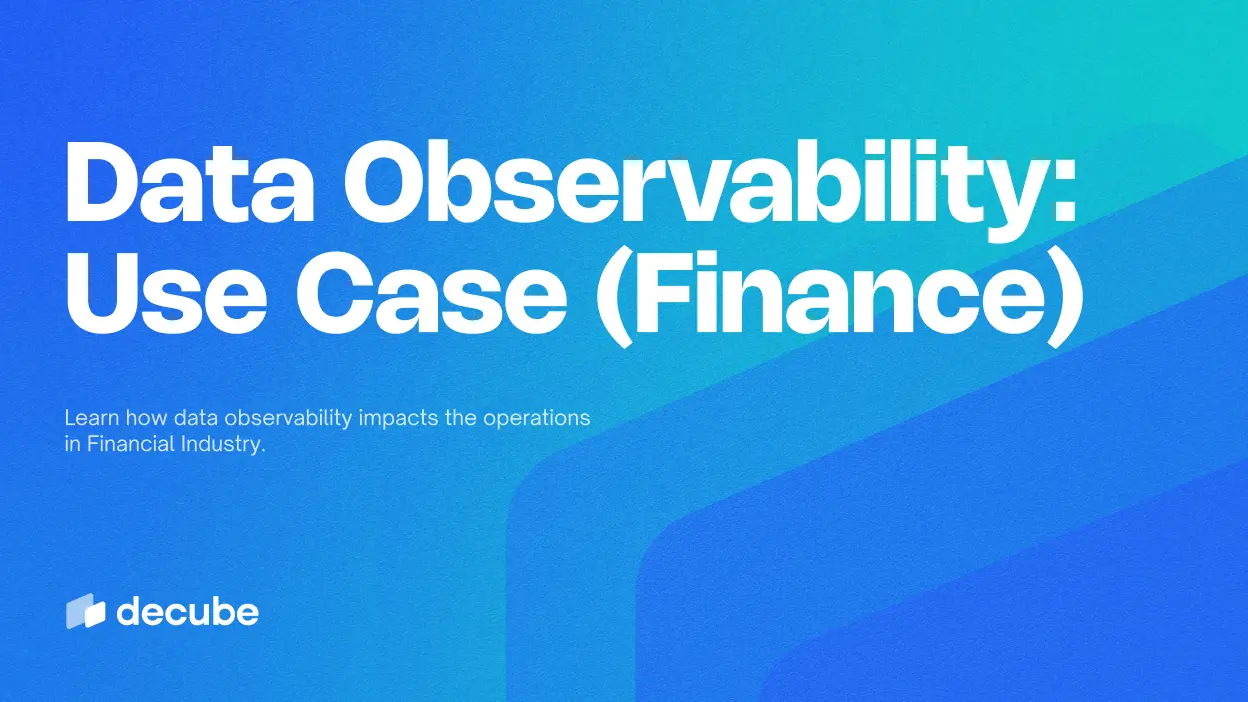
In the fast-paced world of finance, data flows like a river, carrying vital information. Imagine a trading floor at Goldman Sachs, where screens flicker with endless numbers. Suddenly, an anomaly appears – a data point that doesn't quite fit.
In the past, such discrepancies might have gone unnoticed, leading to costly mistakes. But today, thanks to data observability, such issues are caught in real-time. This ensures the integrity of financial operations.
We've entered an era where data observability is key for financial data monitoring. With global digital banking users expected to reach 3.6 billion by 2024, the data volume is staggering. This surge in digital transactions has made regulatory compliance more critical than ever.
Financial institutions must adopt robust data observability practices. This is crucial for their survival in the complex financial landscape.
Consider Checkout.com, which monitors over 4,600 data sets daily. Their use of data observability supports 300+ active data platform users. It also keeps 1,200+ dbt models running various transformations healthy.
This level of oversight is no longer a luxury – it's a necessity in modern finance.
Key Takeaways
- Data observability ensures real-time monitoring of financial data integrity
- Global digital banking users are projected to reach 3.6 billion by 2024
- Checkout.com monitors 4,600+ data sets and supports 300+ active users daily
- Regulatory compliance is driving the adoption of data observability in finance
- Real-time anomaly detection is crucial for preventing costly financial mistakes
Understanding Data Observability in Finance
Data observability in finance helps institutions understand their data systems better. It's a new technology that changes how data engineering works, especially in finance. Here, having accurate data is key.
Definition and Importance
Data observability means watching and analyzing data from start to end. In finance, it's vital for keeping data quality high and reports reliable. General Electric (GE) uses these tools to check financial data in real-time. This makes their reports more accurate for making decisions and following rules.
Key Metrics in Financial Data Observability
Financial places focus on a few important metrics:
- Data freshness
- Volume changes
- Field-level oddities
- Data lineage tracking
These help spot data problems early. This cuts down the time to find issues from 4 hours to just minutes.
Benefits for Financial Institutions
Using data observability brings big benefits:
- Better data reliability
- Quicker problem finding and fixing
- Better monitoring of rules
- Less money spent on operations
Airbnb's "Minerva" tool shows these benefits. It automates checking data quality, saving a lot of manual work. Financial places can cut the time spent on bad data practices from 40% to almost nothing. This saves a lot of money.
By using machine learning for finding oddities and tracking data from start to end, financial places can keep their data top-notch. This approach boosts data reliability and follows rules better. It also makes operations more efficient.
The Rise of Data Complexity in Financial Services
Financial services are seeing a big increase in data complexity. This is due to mobile banking, open financial data, and AI. Now, making decisions based on data is becoming more common in finance.
This change requires better data technology and strong financial data management. The industry is dealing with complex data pipelines. These pipelines link different databases and systems together.
Without careful management, mistakes can happen. Keeping data accurate and reliable across all platforms is key.
These numbers show why data observability is so important in finance. It lets us watch data in real-time for any oddities. This is crucial for stopping fraud and keeping data trustworthy.
Financial institutions are making changes. 38% have now achieved full-stack observability. This is 15% more than other industries.
They're focusing on security, governance, risk, and compliance. 54% of organizations are putting these areas first.
As data complexity grows, so does the need for good data pipelines and management in finance. It's clear that using data to make decisions is now a must for success in this changing world.
Data Observability: Use Case in Financial Industry
Data observability is key in the financial world. It helps in many ways, like watching transactions and making sure rules are followed. Let's see how it changes how finance works.
Real-time Transaction Monitoring
Financial groups use data observability to watch transactions live. This lets them catch odd patterns fast. For instance, PhonePe, a big P2P payments company, handles over 2 billion transactions a month with this tech. It keeps financial systems safe.
Fraud Detection and Prevention
Fighting fraud is a big job for data observability in finance. Banks check transaction data live to find fraud fast. This is important because money laundering is a big problem worldwide, costing 2-5% of global GDP. Data observability tools help banks stay one step ahead of fraudsters and keep customers safe.
Regulatory Compliance Management
Data observability also helps with following rules in finance. It makes sure banks follow laws and avoid big fines. With more global trade, following rules is more important than ever. Data observability keeps banks on track.
Data observability in finance does more than just these things. It also finds insider threats, makes operations better, and cuts down on data costs. Gartner says bad data quality can cost a company over $14 million a year. With data observability, banks can avoid these losses and do better overall.
Implementing Data Observability in Banking Systems
Banking systems are changing fast, with 73% of interactions now online. This change means banks need better data quality and clear audit trails. They're moving from old mainframes to new cloud setups, which are faster but also more complex.
Banks are using data observability tools to handle these changes. These tools check data automatically and are easy to use. They give leaders a clear view of everything. For example, banks use them to keep track of SCRA rules for military members.
- Setting up automated quality assurance measures
- Establishing preventative data health strategies
- Deploying volume management solutions to handle massive data influxes
Neobanks are leading in digital banking, and fintech partnerships help traditional banks offer new services quickly. Data observability is key here. It keeps data quality high and audit trails reliable, which is vital for following rules and keeping customers happy.
"In today's digital-first banking environment, implementing robust data observability is not just a necessity, it's a competitive advantage."
As we move forward in banking, data observability will be crucial. It will help make banking services safe, efficient, and focused on customers.
Enhancing Data Quality and Reliability in Finance
In the financial world, having good data is key. Bad data can cause big losses, with companies losing up to $15 million a year. To fix this, banks and financial firms are using better data management.
Automated Quality Assurance
Automated checks are changing how we analyze financial data. These systems cut down on manual work by up to 60%. This lets companies check their data more easily and make fewer mistakes.
Data Lineage Tracking
Tracking where data comes from is important for keeping data reliable. It shows how data changes from start to finish. This is key for following rules, like those from the Dodd-Frank Act and GDPR.
Anomaly Detection in Financial Data
Using advanced tools to spot odd patterns in financial data is crucial. These tools help find fraud or data problems early. For example, checking over 28,000 currency pairs in foreign exchange keeps data safe.
By working on these areas, financial companies can make their data better and more reliable. This makes their work more efficient and builds trust with others. It also helps them follow the rules.
Data Observability for Risk Management in Finance
Data observability is changing how finance handles risk. It gives real-time views of data quality, helping make risk assessments more accurate and timely. Financial groups use it to watch key risk signs and spot oddities that might mean higher risk.
Poor data quality can hurt risk management a lot. Gartner says bad data costs about $15 million a year. In finance, this can cause big money losses and slow down work.
- Real-time monitoring of risk indicators
- Early detection of data anomalies
- Improved data reliability for risk models
- Enhanced regulatory compliance
More complex financial data and new tech are pushing finance towards data observability. Neobanks and fintech are changing the game, making tools for data-driven risk assessment more important than ever.
With data observability, finance can spot and fix risks before they get big. This makes financial stability and following rules better. It's key in today's complex finance world.
Optimizing Customer Experience through Data Insights
Data observability changes how banks serve customers. Now, banks offer services that fit each person's needs. This is thanks to real-time data analysis of what customers like and do.
Personalized Banking Services
Banks now create products that match what customers want. This means everything from special savings plans to advice on investments. Customers are happier and more loyal because of it.
Improved Customer Support
With data observability, customer info is always right and up-to-date. This makes solving problems faster and service more efficient. Support teams can use detailed customer profiles to help better.
Data-Driven Product Development
Financial institutions make products based on what customers really need. By looking at how people use things and what they say, banks find out what's missing. Then, they make products that really connect with their clients.
- 80% of executives don't fully trust their organization's data
- Data observability can reduce detection and restoration times by up to 60% and 80% respectively
- Predictive analytics and targeted troubleshooting significantly impact the bottom line
These stats show how crucial good data is for better customer service and new product ideas in banking.
Challenges in Adopting Data Observability in Finance
Implementing data observability in finance is tough. Data privacy and security are major concerns. A huge 75% of Chief Information Security Officers (CISOs) in finance say managing vulnerabilities is harder now.
Only 6% of financial companies can see runtime vulnerabilities in real-time. This makes things even harder.
Setting up data observability is also a big challenge. Even though 58% of financial institutions use five or more security solutions, combining them with old systems is tough. The amount of data is overwhelming.
Financial teams deal with over 2,200 alerts about possible vulnerabilities every month. Sadly, 75% of these alerts are false positives. This makes finding real threats very difficult.
Cultural changes are also a big hurdle. Only 37% of financial companies have a strong DevSecOps culture. This means security is not a part of the whole software development process.
To get past these challenges, 82% of CISOs think security should be everyone's job in software delivery. Changing the culture is key to making data observability work in finance.
FAQ
What is data observability in the financial industry?
In finance, data observability means knowing the health and quality of data. It helps organizations find and fix data issues early. This ensures data is reliable, accurate, and consistent.
What are the key metrics tracked during data observability processes?
Important metrics include data quality, how fast it's updated, and if it's complete. In finance, it also looks at data freshness, volume, and any field-level issues.
What are the benefits of data observability for financial institutions?
Financial institutions gain from better data reliability and quicker issue solving. They also get better at following rules and reduce data downtime.
How does data observability aid in real-time transaction monitoring and fraud detection?
It helps by analyzing transactions in real-time. This way, it spots suspicious patterns or fraud. It keeps financial transactions safe and sound.
How does data observability support regulatory compliance management?
It ensures financial data meets anti-money laundering (AML) rules and other standards. This is through thorough monitoring and analysis.
What are some key practices in implementing data observability in banking systems?
Key practices include using automated data checks and setting up strategies to keep data healthy. It also involves managing data volume and using easy-to-use interfaces for full visibility.
How does data observability enhance data quality and reliability in finance?
It uses automated checks, tracks data lineage, and spots anomalies. This helps scale data validation, trace data changes, and find data quality issues.
What role does data observability play in risk management for financial institutions?
It gives real-time insights into data quality and integrity. This leads to more accurate and timely risk assessments. It helps monitor key risk indicators and ensures data reliability in risk models.
How can data observability optimize customer experience in the financial industry?
It ensures customer data is accurate and reliable. This leads to better personalized services, improved support, and data-driven product development. It meets customer needs better.
What are some challenges in adopting data observability in finance?
Challenges include protecting data privacy and security. It also involves integrating tools with old systems, managing huge data volumes, and changing the data culture.