Kindly fill up the following to try out our sandbox experience. We will get back to you at the earliest.
Data quality management : Tips & Best practices for reliable data
Learn tips and best practices for ensuring reliable data through data quality management, including standards, audits, validation, and governance.
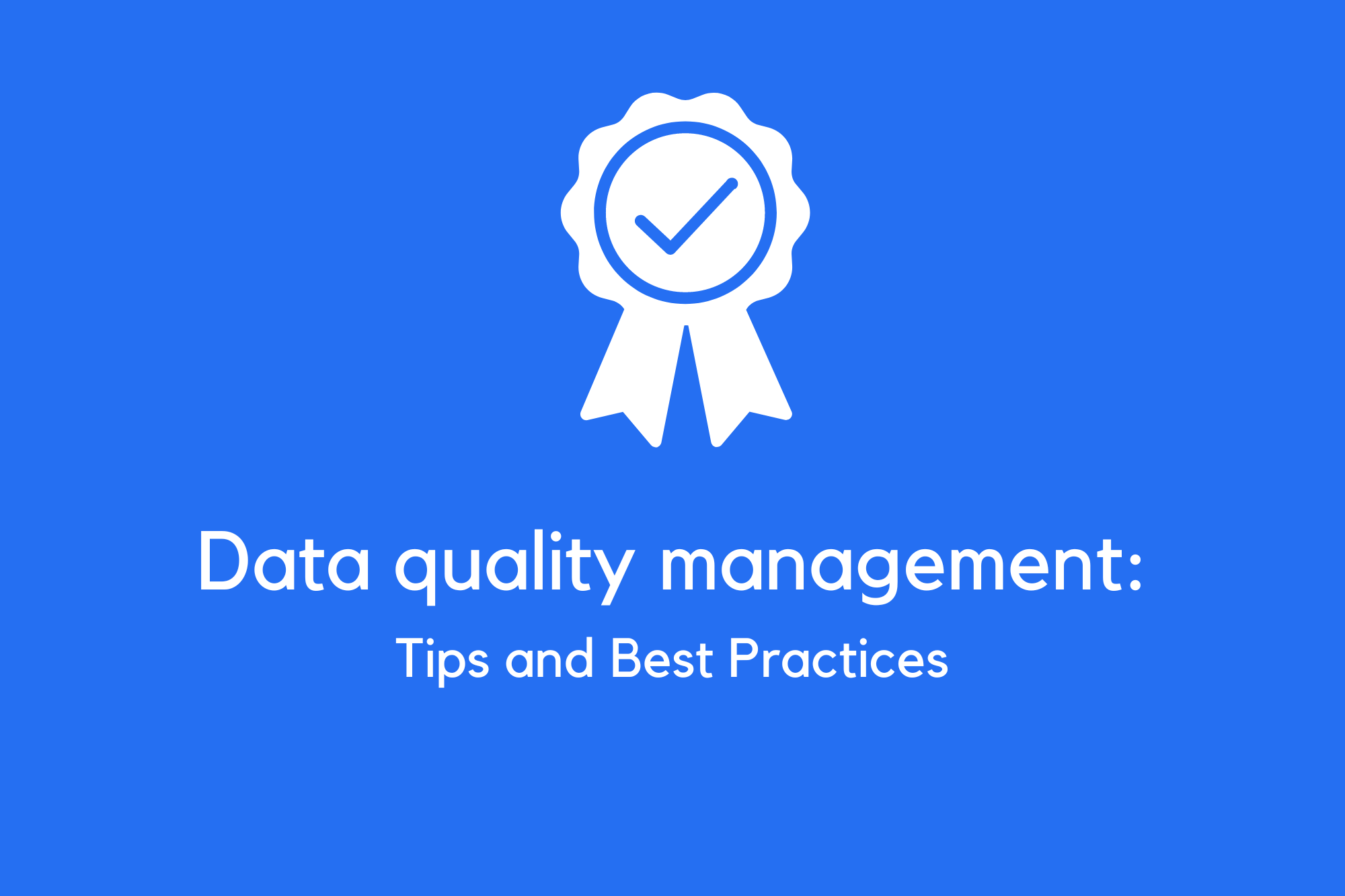
As a mid-sized company, the thought of managing your organization's data quality effectively is a dreadful thought that keeps any data officer uneasy. With the abundance of data being generated and collected, ensuring that your data is accurate, complete, and consistent is becoming increasingly important. But how do you achieve this goal?
Luckily, several data quality tools and technologies have emerged in the market to help organizations manage their data quality effectively. Today's blog will equip you with the knowledge and skills to manage data quality effectively. So, let's get started!
Common Data Quality Issues and How to Address Them:
But what is it with data? And when does data become useless? Data quality issues can arise from various sources, like human error, system limitations, and changes in business requirements. So when do we say there is a data quality issue?
1. Duplicate Data: This is one of the most common data quality issues resulting from manual data entry errors, data imports, or data synchronization issues. Duplicate data can cause inaccuracies in reporting, as well as incur unnecessary storage costs.
Organizations can implement de-duplication strategies to address duplicate data, which involve identifying duplicate data and consolidating it into a single, accurate record. De-duplication can be performed manually or using automated tools. The simplest way is to establish data quality standards and policies right in the beginning to prevent duplicate data from being created in the first place.
2. Incomplete Data: This is the data that is missing critical information required for analysis or decision-making resulting again from manual data entry errors, data source limitations, or system integration issues.
To address incomplete data, organizations can establish data quality standards and policies that require data completeness before it can be entered into the system. It is like a security guard allowing only the relevant data set. This is possible with Data validation rules that can be implemented to ensure that data entered into the system contains all required fields. Automated data quality tools can also help identify and flag incomplete data, enabling organizations to take corrective action.
3. Inaccurate Data: It is erroneous data, such as incorrect values or incorrect formatting resulting from manual data entry errors, data source limitations, or system integration issues.
To address inaccurate data, the above solutions, i.e., having standard policies and implementing data validation rules, will arrange to have accurate data. Automated data quality tools can also help identify and correct inaccurate data, enabling organizations to maintain data accuracy.
4. Outdated Data: It is data that is no longer relevant or up-to-date, resulting from changes in business requirements, system limitations, or data source limitations. Outdated data can cause inaccuracies in reporting and, ultimately, decision-making.
To address outdated data, it is not only important to have standard policies in place, but it is also important to regularly update them. Data validation and tools are consequently important.
5. Inconsistent Data: It is data that vary in format or structure from manual data entry errors or system limitations, making it difficult to compare or analyze.
To address inconsistent data, organizations can establish data quality standards and policies that require data consistency before it can be entered into the system. Apart from data validation techniques, automated data quality tools can also help identify and correct inconsistent data, enabling organizations to maintain data consistency.
Establishing data quality standards and policies is important, such as implementing data validation rules, using automated data quality tools, and assigning data quality responsibilities. And we are aware that clean and accurate data is a gold mine!
Best Practices for Data Quality Management: Tips and Strategies:
Implementing best practices for data quality management can help you maintain what it needs to have clean and reliable data. Some best practices discussed above are having standard policies, strict gatekeeping, data validation, and automated tools to flag any issue. But how do we implement these important things while setting up data quality management measures?
1. Establish Data Quality Standards and Policies: Establishing data quality standards and policies is the first step to ensuring data quality. This involves defining what constitutes good quality data, as well as setting standards for data entry, validation, and storage. A clear and comprehensive set of data quality standards and policies will help ensure that everyone in the organization is aware of what is expected of them when it comes to maintaining data quality.
An organization might establish a data quality policy that requires all new customer data to be validated against existing customer records before being added to the database. This policy can help prevent duplicates and ensure that all customer data is accurate and up-to-date.
2. Perform Regular Data Quality Assessments: We saw that performing regular data quality assessments is critical to identifying and addressing data quality issues. These assessments can help you identify patterns of errors or inconsistencies in your data, as well as pinpoint areas where data quality standards and policies are not being followed. Regular assessments also provide insight into the effectiveness of your data quality management processes, enabling you to make improvements as needed.
For example, an organization might conduct a data quality assessment that involves analyzing customer data to identify any duplicate records or inconsistencies in data formatting. Based on the assessment results, they can take corrective action to ensure that all customer data is accurate and consistent.
3. Use Automated Data Quality Tools: Automated data quality tools can help streamline the data quality management process by automating data profiling, validation, and cleansing tasks. These tools can help ensure that data quality standards and policies are consistently enforced across the organization and can significantly reduce the time and effort required to maintain data quality.
For example, an organization might use a data quality tool such as Trifacta to automate the process of data profiling and validation. This tool can help identify and correct data quality issues in real-time, enabling the organization to maintain data accuracy and consistency.
4. Provide Data Quality Training: Providing data quality training to employees is essential to ensure that data quality standards and policies are followed consistently across the organization. Data quality training should cover topics such as data entry, validation, and storage, as well as the importance of maintaining data quality.
For example, an organization might provide data quality training to new employees as part of their onboarding process. This training could include information on how to enter data accurately and consistently, as well as how to validate and correct data as needed.
5. Assign Data Quality Responsibilities: Assigning data quality responsibilities to specific individuals or teams can help ensure that data quality standards and policies are consistently enforced across the organization. These individuals or teams can be responsible for tasks such as data entry, validation, and storage, as well as monitoring data quality and taking corrective action as needed.
Say, an organization might assign data quality responsibilities to a dedicated data quality team that could be responsible for tasks such as monitoring data quality, identifying data quality issues, and implementing corrective action to maintain data accuracy and consistency.
In summary, establishing data quality standards and policies, performing regular data quality assessments, using automated data quality tools, providing data quality training, and assigning data quality responsibilities are all essential best practices for data quality management. These best practices, if implemented, can go a long way.
Data Quality Tools and Technologies: An Overview:
Data quality issues can arise due to a variety of reasons, such as human error, system failures, or data integration challenges. Data quality tools and technologies are critical for managing clean and reliable data. Technology has made several tools available that have emerged in the market to help organizations with data quality management. Let us see what are these tools:
- Data Quality Management Tools: Using these tools can aid in upholding the precision, comprehensiveness, uniformity, and dependability of your data. Examples of such tools include Decube, Trifacta, Talend, and Informatica, which offer data profiling, validation, cleansing, and standardization capabilities.
- Master Data Management (MDM) Tools: What if you have data stored across multiple systems and applications and need to ensure consistency across all of them? This is where Master Data Management (MDM) tools come into play. MDM tools can help organizations manage master data across multiple systems and applications. Examples of MDM tools include IBM InfoSphere MDM, Informatica MDM, and Talend MDM, which provide data governance, data stewardship, and data integration capabilities.
- Data Integration Tools: Data integration tools can help organizations integrate data from disparate sources and ensure data consistency. Examples of data integration tools include Apache Nifi, IBM InfoSphere DataStage, and Talend, which offer data mapping, data transformation, and data loading capabilities.
- Business Intelligence (BI) Tools: BI tools can help organizations analyze and visualize data to make informed decisions. Examples of BI tools include Tableau, Power BI, and QlikView, which provide data visualization, reporting, and dashboarding capabilities.
In spite of all these tools, it is vital to select the right one that fits your organization's requirements and budget and regularly assess the effectiveness of your data quality practices to ensure that your data is clean, reliable, and trustworthy.
Prioritizing Data Quality Management: A Must for Organizations
The massive inflow of data from various sources makes maintaining data quality a daunting task for many organizations. Yet, it is crucial to acknowledge that overlooking data quality can lead to incorrect business decisions, revenue losses, and negative impacts on brand reputation.
Therefore, organizations must prioritize data quality management by implementing the best practices and strategies discussed in this blog since investing in data quality management is investing in the success of your business. Decube offers data management solutions to help businesses streamline their data processes and improve data quality. Signup today!