Kindly fill up the following to try out our sandbox experience. We will get back to you at the earliest.
What is Machine Learning Data Catalog?
Step into the future with Machine Learning Data Catalogs: Uncover how they streamline data discovery and management for AI and ML projects efficiently.
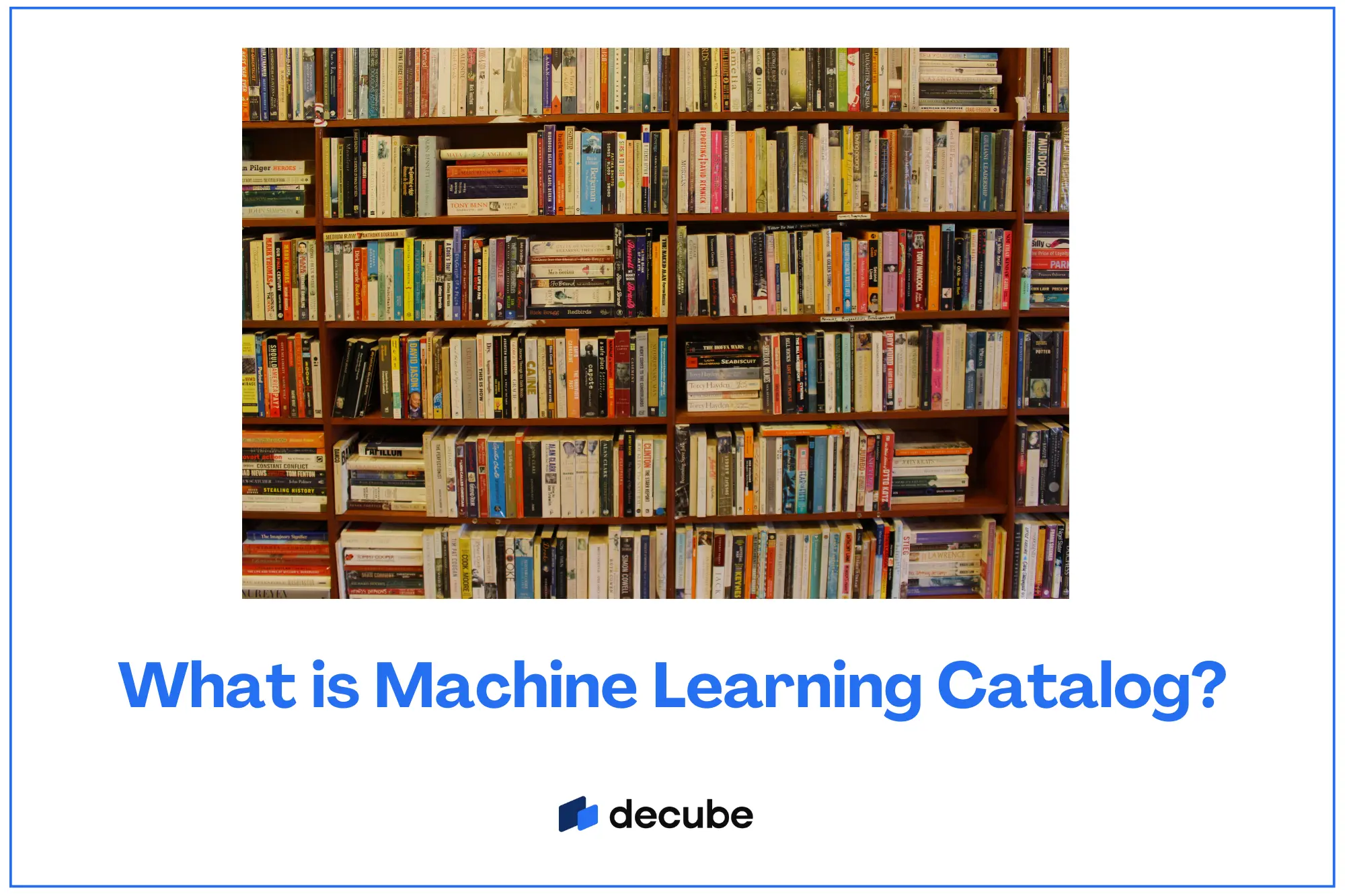
Data is a crucial asset for organizations and is essential for business success. But with the increase of data in this decade, managing and Catalog data has become increasingly complicated and challenging. When we are talking about data Catalog, machine learning has emerged as a powerful tool for data Catalog and metadata management. By automating key tasks and providing greater accuracy and efficiency, machine learning has the potential to revolutionize the way organizations manage their data assets.
In this blog, we’ll learn about machine learning in detail for data catalog and metadata management. We’ll discuss key applications and benefits for organizations looking to extract greater value from their data.
Data Catalog and Metadata Management:
Data Catalog and metadata management are essential components of modern data management methods. Data Catalog involves creating an inventory of the data assets available to an organization, while metadata management involves defining and managing the descriptive information about those assets. This information includes data definitions, relationships, and dependencies, among other things. Data Catalog and Metadata Management are essential for organizations to be able to find, understand, and effectively use their data assets.
Why do you need machine learning in metadata management?
Metadata management provides valuable information about the features, location, and use of data, allowing organizations to make more informed decisions and extract more excellent value from their data. How? You will know shortly! But metadata management can be complex and time-consuming, if done manually, particularly in large organizations with vast amounts of data.
This is where machine learning (ML) comes in!
The Challenges of Manual Data Catalog and Metadata Management:
Manual data Catalog and metadata management can be a time-consuming and error-prone task for organizations and comes with several challenges:
Inconsistency in metadata: Inconsistent metadata can cause confusion and errors when data is used. Different people may use different terms to describe the same data, leading to duplicate entries or missed opportunities to leverage data assets. Manual metadata management can make it difficult to ensure consistency across all data assets, leading to a lack of confidence in the accuracy and reliability of the metadata.
Time-consuming: Manually Catalog and tagging data can take up a lot of time, particularly when dealing with enormous data leading to delays in making data available for analysis or decision-making.
Human error: Manual metadata management is prone to human error. People may misspell or mistype metadata tags, leading to inaccurate data classification or missed opportunities to leverage data assets.
Limited scalability: Manual metadata management is not scalable. As the magnitude of data increases, the time and effort required to manually tag and catalog data also increases making it difficult for organizations to keep up with the pace of data growth and can limit their capacity to extract value from their data assets.
Compliance risks: Many organizations are subject to regulatory requirements that mandate accurate and up-to-date metadata for their data assets. Manual metadata management can increase the risk of non-compliance, leading to fines, legal action, and damage to the organization's reputation.
Machine learning can help organizations overcome these challenges by automating key tasks and providing greater accuracy and consistency in metadata management by enabling them to extract greater value from their data assets.
So, How Machine Learning Can Improve Data Catalog and Metadata Management:
Machine learning (ML) can help organizations address the challenges of manual data Catalog and metadata management.
Key Applications of Machine Learning in Data Catalog and Metadata Management:
Machine learning (ML) has several key applications in data Catalog and metadata management. These applications can help organizations overcome the challenges of Manual data Catalog by automating key tasks, improving accuracy and consistency, and enhancing compliance and governance. Some of the key applications of ML are:
1. Metadata extraction and tagging: ML algorithms can be used to automatically extract metadata from data sources and tag the data with the appropriate metadata tags. This can save time and reduce errors associated with manual metadata tagging. For example, ML algorithms can be trained to recognize patterns in data and automatically generate metadata tags based on those patterns.
2. Data profiling and schema inference: ML algorithms can be used to automatically profile data sources and infer their underlying schemas. This can help organizations gain a better understanding of their data assets and ensure that metadata is consistent and accurate across all data sources. For example, ML algorithms can be trained to identify relationships between data elements and automatically infer the underlying data schema.
3. Data quality assessment: ML algorithms can be used to automatically assess the quality of data sources and identify potential data quality issues, such as inconsistencies or errors in metadata. This can help organizations maintain high standards of data quality and ensure that data is used appropriately and ethically.
4. Data classification and clustering: ML algorithms can automatically classify and cluster data sources based on their metadata tags. This can help organizations gain a better understanding of their data assets and identify relationships between different data sources.
5. Data lineage tracking: ML algorithms can be used to automatically track the lineage of data sources and metadata tags, enabling organizations to understand how data has been transformed and used over time. This can help organizations maintain a comprehensive understanding of their data assets and ensure compliance with regulatory requirements.
The Role of Machine Learning in Data Profiling and Schema Inference:
Machine learning (ML) can play a vital role in data profiling and schema inference, which are important elements of metadata management. Data profiling involves analyzing data sources to understand their content, quality, and structure, while schema inference involves using this information to automatically generate data schemas.
ML can help organizations improve data profiling and schema inference in several ways, including:
Automatic data profiling: ML algorithms can be trained to automatically profile data sources, enabling organizations to analyze large datasets quickly and efficiently. For example, ML algorithms can be used to identify data types, detect data anomalies, and assess data quality.
Automatic schema inference: ML algorithms can be used to automatically generate data schemas based on data profiling results, eliminating the need for manual schema creation. This can save time and reduce errors associated with manual schema creation, particularly for large or complex datasets.
Schema evolution: ML algorithms can be used to automatically update data schemas as new data sources become available or as data sources change over time. This can help organizations maintain accurate and up-to-date data schemas, improving data consistency and enabling effective data management.
Data classification: ML algorithms can automatically classify data sources based on their content and structure, enabling organizations to manage and protect sensitive data more effectively which helps organizations comply with data privacy regulations and reduce the risk of data breaches.
Data discovery: ML algorithms can be used to automatically discover new data sources and add them to existing metadata catalogs, enabling organizations to improve their understanding of their data assets and uncover new opportunities for analysis and insights.
Enriching Data Governance and Compliance with Machine Learning:
Enhancing data governance and compliance is a critical part of metadata management, and machine learning (ML) can play a powerful role in achieving these goals. ML algorithms can help organizations improve data governance and compliance in several ways, including:
Data classification: ML algorithms can be used to automatically classify data sources based on their sensitivity, enabling organizations to manage and protect sensitive data more effectively. This helps organizations comply with data privacy regulations, such as GDPR or CCPA, and reduce the risk of data breaches.
Data lineage tracking: ML algorithms can be used to automatically track the lineage of data sources and metadata tags, enabling organizations to understand how data has been transformed and used over time. This can help organizations maintain a comprehensive understanding of their data assets and ensure compliance with regulatory requirements.
Anomaly detection: ML algorithms can be used to detect anomalies in data sources, such as outliers or unexpected patterns, which may indicate data quality issues or potential compliance violations. This can help organizations identify and address compliance issues more quickly, reducing the risk of penalties or fines.
Access control: ML algorithms can be used to automatically monitor data access and usage patterns, enabling organizations to detect and prevent unauthorized access to sensitive data.
Risk assessment: ML algorithms can be used to automatically assess the risk associated with different data sources, enabling organizations to prioritize metadata management efforts and allocate resources more effectively. This can help organizations reduce the risk of compliance violations and improve data governance.
The Benefits of Machine Learning for Data Catalog and Metadata Management:
Machine learning has transformed the mode we manage and analyze data, and its impact on metadata management cannot be exaggerated. From automating metadata extraction and tagging to improving data governance and compliance, ML has the potential to streamline metadata management tasks and unlock new insights from data.
ML will become an increasingly important tool for metadata management, enabling organizations to unlock the full potential of their data assets.
So, if you want to stay ahead in this competitive world and harness the full potential of your data assets, consider integrating machine learning into your metadata management strategy today.
External Reference:
- TechTarget has an article on Why you should consider a machine learning data catalog which explains how machine learning data catalogs can benefit an enterprise in a variety of ways, from increasing access to necessary data to keeping your data sources up to date.
- G2 has a list of Best Machine Learning Data Catalog Software which explains how machine learning data catalogs allow companies to categorize, access, interpret, and collaborate around company data across multiple data sources while maintaining a high level of governance and access management.
- Express Analytics has an article on What Is Machine Learning Data Catalogs? Benefits and Use-cases which explains how machine learning data catalogs provide the best possible way of managing, monitoring, and improving the use of enterprise data assets. They can be of great benefit especially to those companies working in heavily regulated industries such as healthcare, finance, and defense.