Kindly fill up the following to try out our sandbox experience. We will get back to you at the earliest.
Reduce Time to Value for Data Observability
Discover how our revamped Config module speeds up data observability implementation, helping organizations achieve quick and efficient results.

Many data observability solutions on the market today require manual writing of tests and their individual test settings, which can make it time-consuming to get started. This can be a barrier for organizations with large number of data sources and assets that are looking to quickly implement data observability and start reaping the benefits of improved data quality.
As a result of these challenges, it can take weeks or even months for organizations to implement data observability and start seeing the benefits. The good news is that in the new generation of data observability solutions, the setup process of monitoring does not need extensive manual configuration. Features that aid the process of quick setup time are pre-built tests, auto-threshold settings for monitoring with intelligent ML models and the ability to pre-train monitors with historical data to shorten the training period.
Our platform now supports all of these, plus more in this feature-packed update which we’ve rolled into a new module called the Config. This is the first article of a two-part update on the Config.
Unified Platform, Unified Monitoring
One of the most popular feature requests from our users is the ability to access and manage all monitors from one screen, so that they can have a very quick overview of what checks are running in the moment. So we introduced two things: A landing page to set up monitoring where you are led into the quick set up process for each monitor, and also one page where you can see all active monitors in your account.
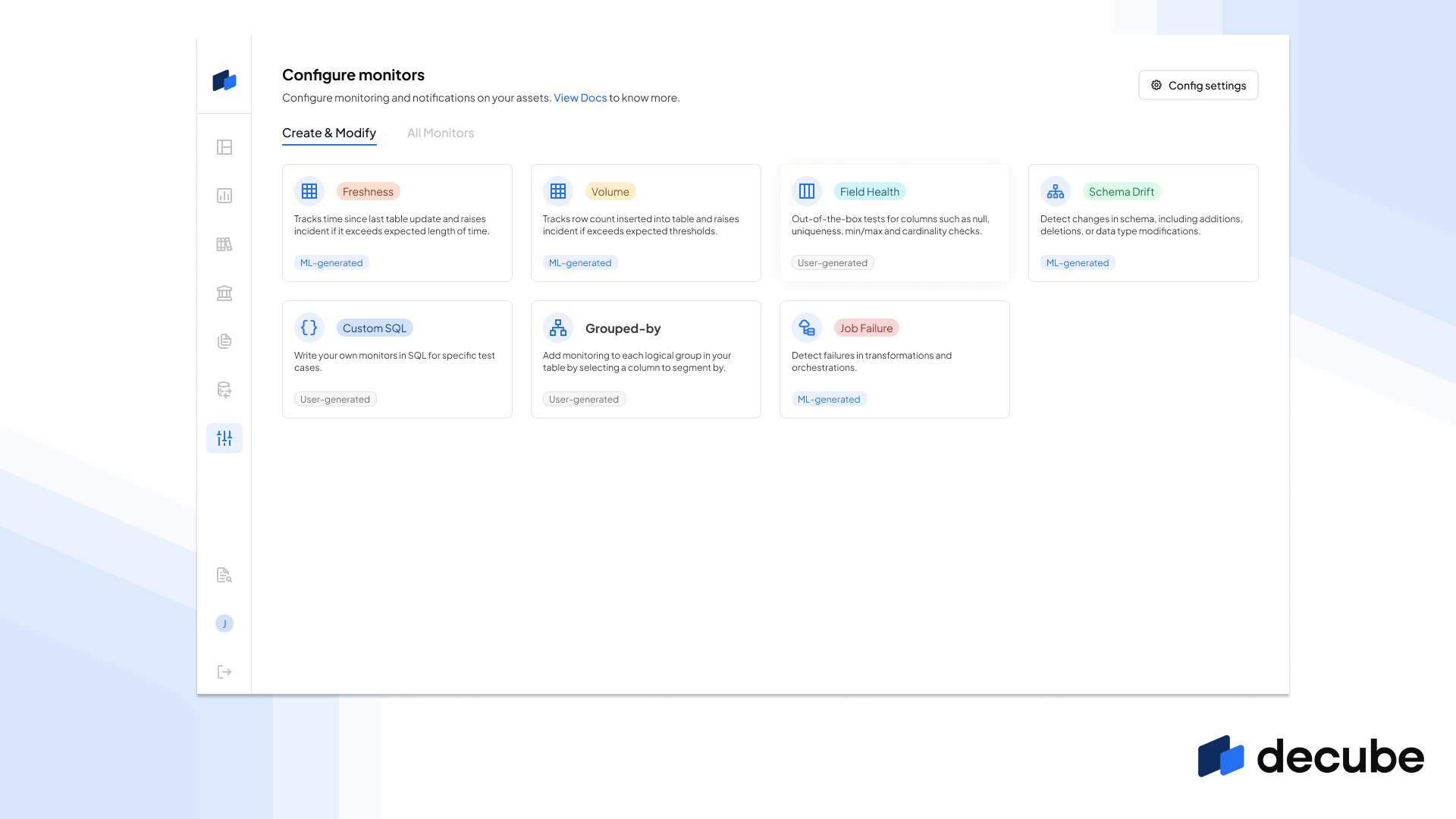
The All monitors page is a great way to have a quick look and filter the active monitors based on their statuses, types of tests being run and the data source. This can help you to quickly identify any monitors that are failing or that need attention.

Define Incident Levels for each monitor
A job failure for some transformation jobs you’re monitoring in dbt may be a lot less important than the few you actually want to pay attention to. Now, for each monitor you’ve set up in your data source, you can set different incident levels for each monitor. With levels such as “Info”, “Warning” and “Critical”, you get incidents in decube and to your notifications with the appropriate incident level so you can quickly judge the priority of attending to a specific incident.

More Control on Schema Drift tests
When you connect your data source, we’re already turning on the monitoring for your schemas to ensure full coverage on any schema change type that may cause downstream failure. However, we’ve made it even better by allowing you to define which schema change type that you’ll want to alert you, and also disable any schema that you would not need to be monitored.
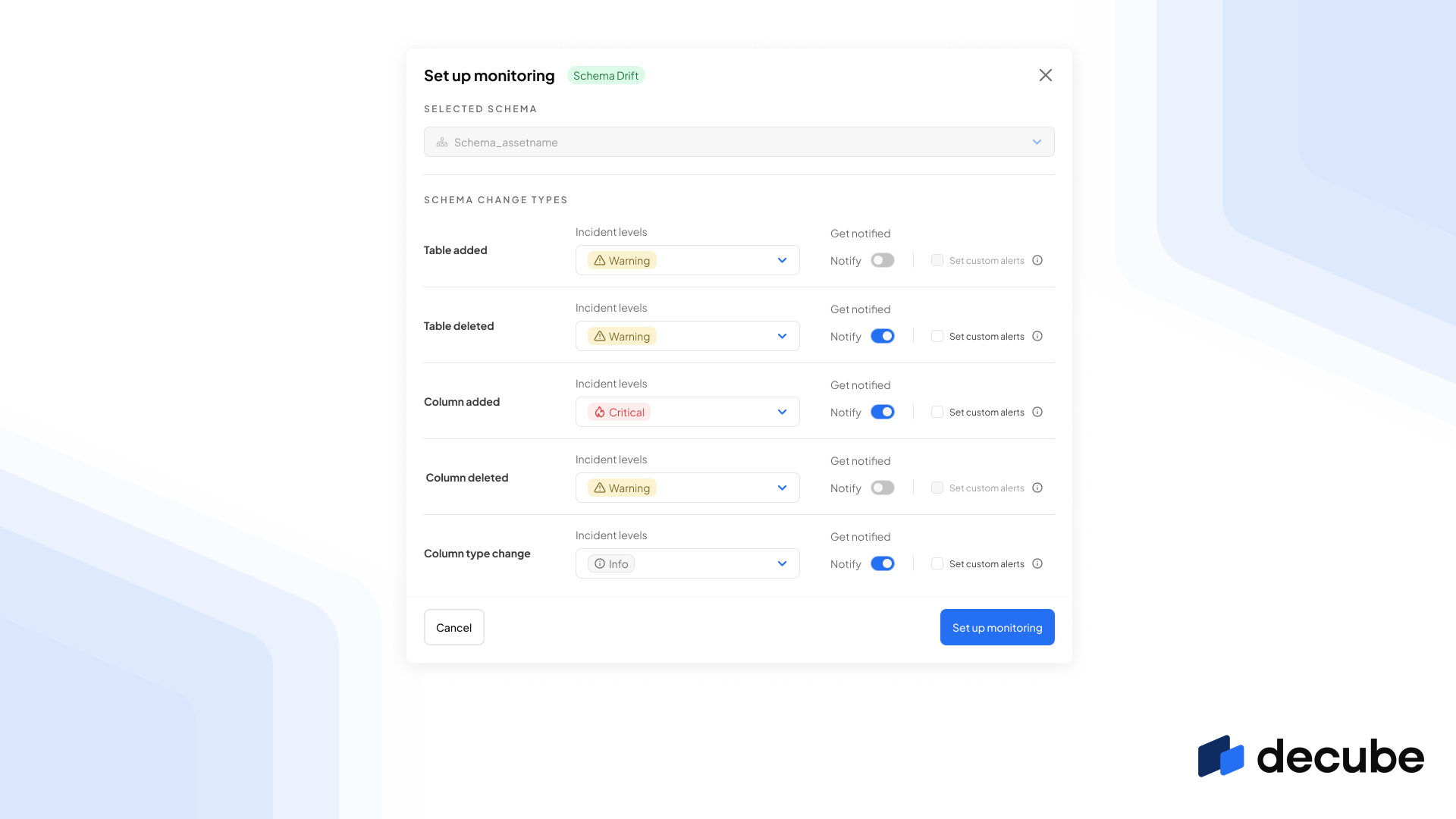
So, you can set table addition of less importance by only defining it as “Info” and not get alerted on it, while setting column deletions at “Warning” for certain schemas.
Bulk Addition of Field Health tests
Instead of needing to add one test to one column at a time, we now support the bulk addition of a test type across multiple fields. This helps you to add coverage of our out-of-the-box tests like null, duplicate and cardinality tests and apply the same settings across to multiple columns in a table. This saves you a significant amount of time, especially if you have a large number of columns to test.

This works well with our “Enable Machine Learning” feature where instead of defining manual thresholds, you can use this to let our ML model calculate the thresholds based on yours scanned metrics.
Alert notifications that matter to who receives it
From a day to day basis, teams get bombarded with alerts from different tools all the time, which interrupts the focus time one needs and attend to the alert. So, one of the significant changes to our alert system is the ability to send individual custom alerts to those who would need to receive it.
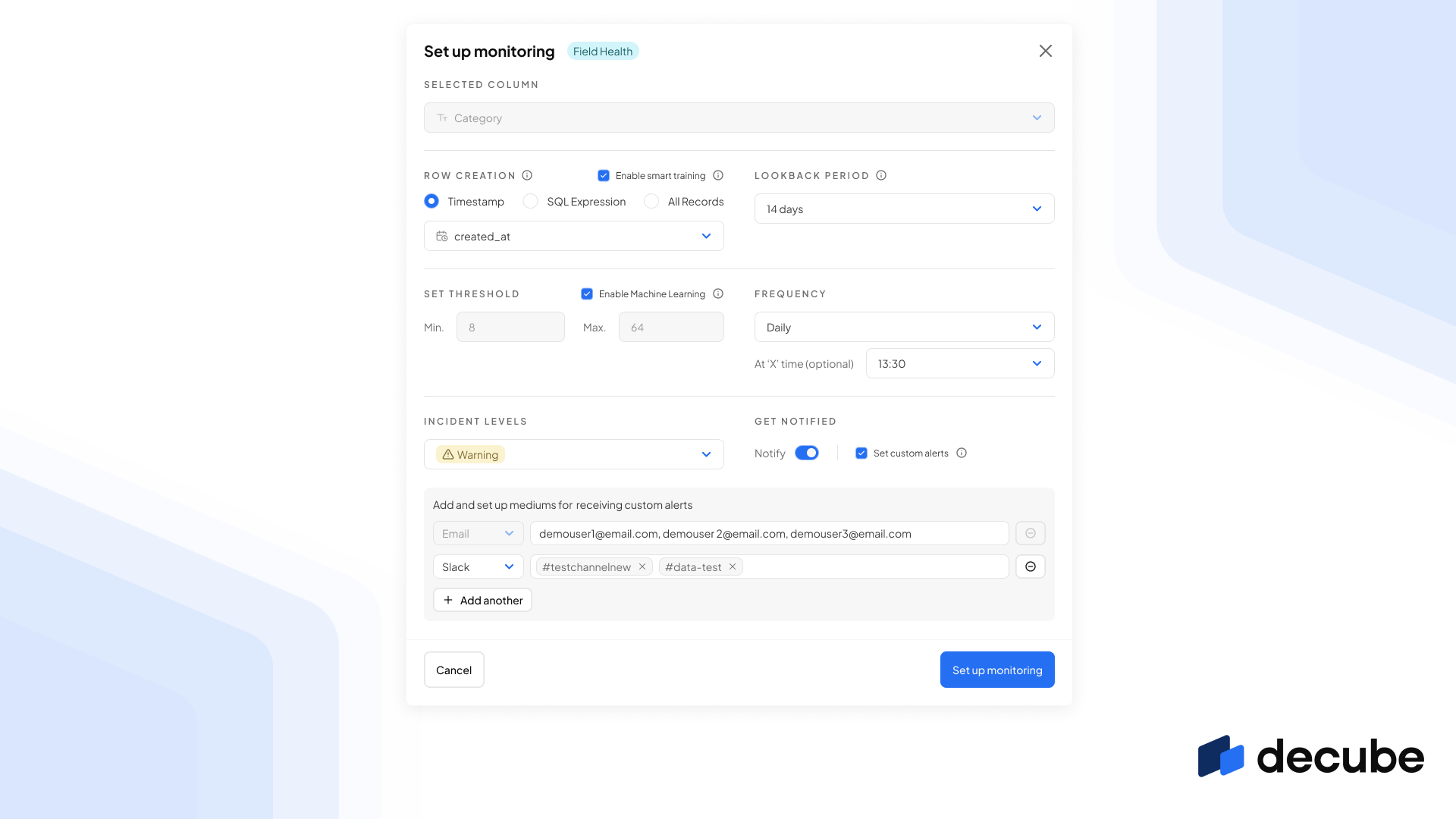
For example, for Airflow job failures, you may want to send it directly to a #job-failures Slack channel where there’s a limited audience of engineers who are able to access and troubleshoot on Airflow. You can do this by heading into any monitor and set custom alerts and input the channel name directly.

Each alert notification has been improved as well to also include a link directly to our new Incident Details which provides a lot of information on the incident trend, and metrics from the failed test that generated the incident.
Optimize the training period for your monitors
When you start a monitor, it usually takes about 3-5 days depending on the frequency of your table updates to generate the ML model for thresholding before the first incident is triggered. However, if you enable our smart training capability, you’ll be able to reduce this training period significantly, as the monitor is able to scan historical data on your table and generate the model for the thresholds, and start triggering incidents early.
If you enable a lookback period (eg. 7 days, 14 days and 30 days), you’ll also be able to see the scanned metrics in the Asset Details for the period that you had enabled the lookback on, and check whether there were any anomalies in your data before you enabled the monitoring on it.
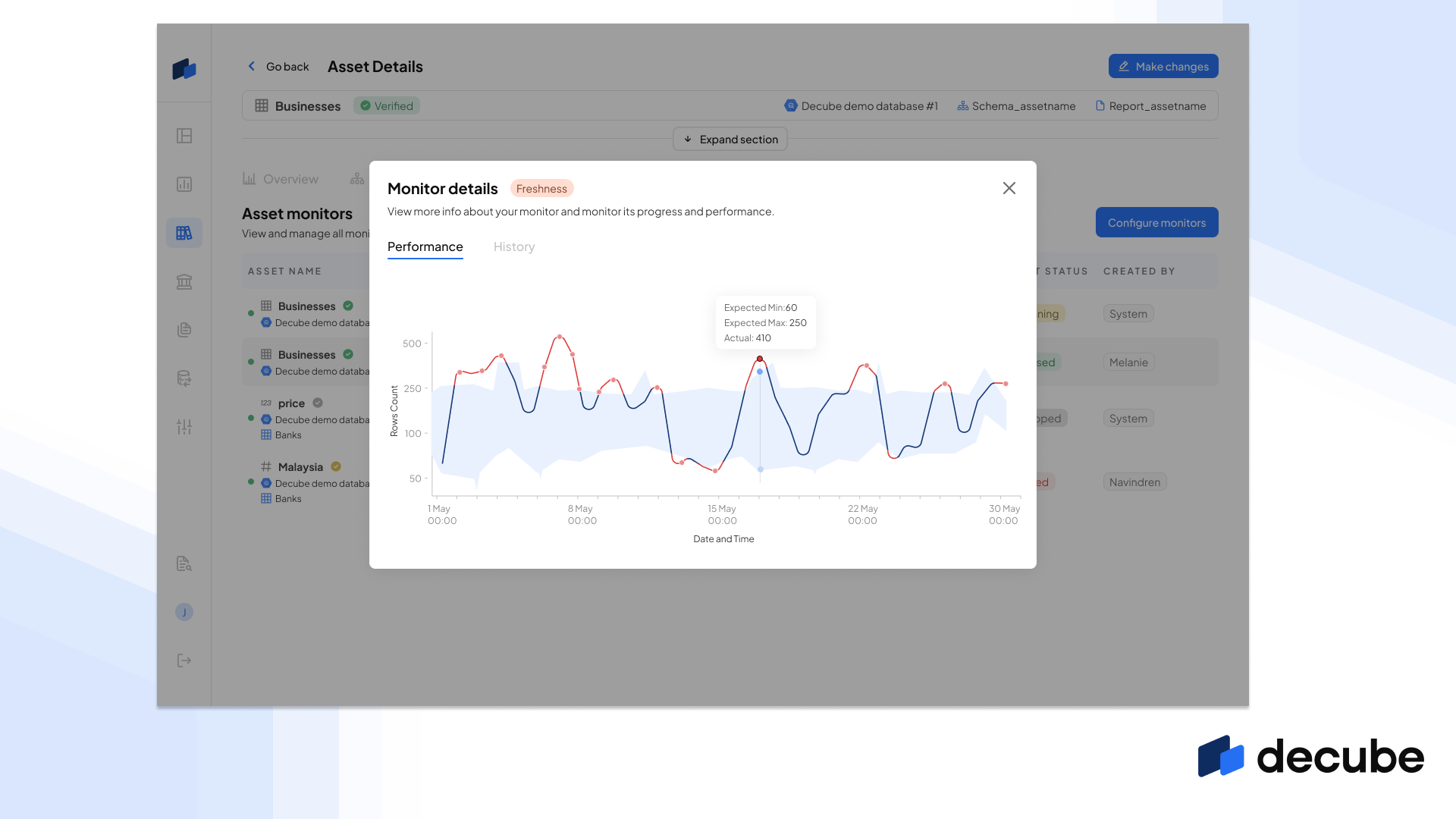
Check out the updates today.
All the above updates were rolled out to our users this week. If you’ll like to see it for yourself, you can sign up for a 30 day trial or request for access to our sandbox account here.
What’s next? Here’s a sneak peek for the second part of our Config update: I’ll be talking about a new type of monitor that we’ve added to our platform. To stay up to date, follow our Linkedin page.